Towards Trustworthy Control for Networked Systems in the AI Era
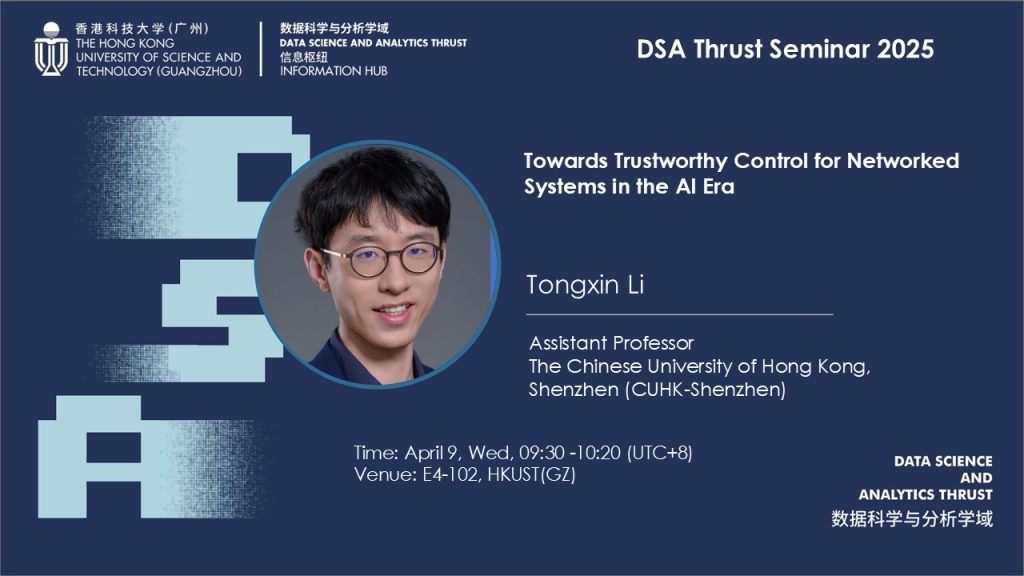
摘要
In today’s AI-driven landscape, managing large-scale networked systems such as power grids, transportation networks, or communication infrastructures requires new control strategies that balance performance with reliability. AI offers powerful tools, like predictive models, to enhance decision-making in these systems. However, it also introduces challenges of uncertainties in predictions. This talk presents ways to designing distributed controllers that integrate AI-driven predictions while ensuring trustworthy operation. We begin with an overview of classic results, such as the Linear Quadratic Regulator (LQR) with predictions. Then, we introduce PredSLS, a framework that synthesizes closed-loop controllers using an affine feedback structure that combines real-time state feedback with future predictions for distributed control. A critical challenge emerges from prediction errors of AI tools, which can propagate through the network and degrade performance, particularly in decentralized settings. Unlike existing methods that often assume perfect predictions, our approach explicitly accounts for these inaccuracies. Through theoretical analysis and empirical studies, we identify an optimal degree of decentralization as a "sweet spot" where the system balances the advantages of local data sharing with the need to minimize the impact of prediction errors. Through this talk, we will demonstrate using real-world examples to how to deliver scalable, robust, and computationally efficient control, addressing the trust challenges posed by AI in networked systems.
演讲者简介
日期
09 April 2025
时间
09:30:00 - 10:20:00
地点
E4-102(HKUST-GZ)
联系邮箱
dsarpg@hkust-gz.edu.cn