Bayesian Oceanographic Flow Cytometry Data Analysis and Mixed-input Gaussian Process Regression
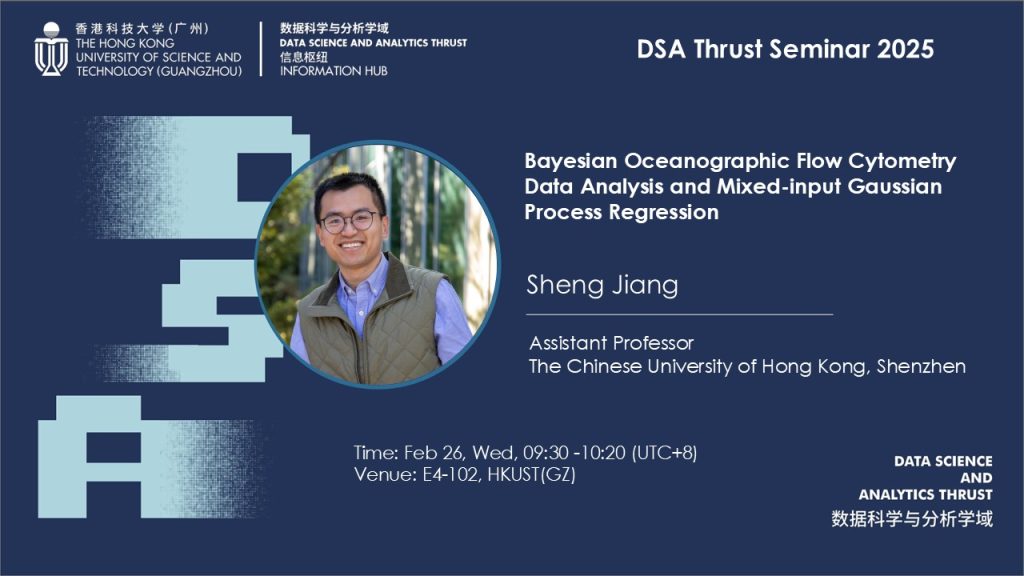
摘要
In this talk, I will present two projects.
The first project is a hierarchical extension of the Bayesian mixture-of-experts model for the censored oceanographic flow cytometry data. This work aims to enhance our understanding of the relationship between marine microbial populations and environmental factors. The dynamics of marine phytoplankton and their relationship with the ocean environment are fundamental to oceanography and our planet. Recent technological advancements have enabled the massive collection of oceanographic flow cytometry data in real-time onboard moving ships. However, instrument limitations confine the data to a restricted region, causing observations originally outside this region to bunch at the boundary. To address this, our Bayesian method imputes the censored data within a Gibbs sampler. Beyond identifying key environmental drivers, our model provides a natural uncertainty assessment of the time-varying relative abundance of phytoplankton populations.
The second project focuses on a latent variable model for mixed-input Gaussian process (GP) regression. Specifying an appropriate covariance kernel is crucial for Gaussian Process (GP) regression. However, selecting an optimal kernel remains challenging when dealing with both quantitative and qualitative (Q\&Q) inputs. We propose a novel latent variable approach which models the qualitative inputs as functions of latent numerical inputs. By imputing the qualitative inputs into numerical ones, the mixed-input GP regression problem reduces to a standard GP regression with only numerical inputs, allowing for flexible and well-understood kernel choices. The latent variable model unifies many influential methods in the literature; it immediately improves computer emulators with Q\&Q inputs in prediction accuracy and uncertainty quantification, as well as downstream applications, such as mixed-variable Bayesian optimization and inverse problems. This paper also develops an efficient Markov Chain Monte Carlo algorithm for sampling from the posterior distribution and making predictions.
演讲者简介
Sheng Jiang is currently an Assistant Professor (tenure-track) in the School of Data Science, Chinese University of Hong Kong, Shenzhen. His research interests primarily lie in Bayesian nonparametrics, encompassing both theoretical and computational aspects, as well as applications to real-world data. His goal is to develop novel methods for understanding large, complex datasets in scientific applications, striking a balance between modeling flexibility and scientific interpretability. Specifically, he has been working on Bayesian nonparametric methods with Gaussian process priors,variable/model selection, variational Bayes, Bayesian stochastic block models, and oceanographic flow cytometry data analysis. His previous work on Bayesian nonparametric methods with Gaussian process priors has been published in leading statistics journals such as AOS and JASA. Before moving to Shenzhen, he served as a Visiting Assistant Professor in the Department of Statistics at the University of California, Santa Cruz for 2.5 years. He also spent one semester as a post-doctoral associate at Duke University, co-advised by Surya Tokdar and Alexander Volfovsky. He completed his Ph.D. in Statistics at Duke University under the supervision of Surya Tokdar.
日期
26 February 2025
时间
09:30:00 - 10:20:00
地点
香港科技大学(广州)E4-1F-102
主办方
数据科学与分析学域
联系邮箱
dsarpg@hkust-gz.edu.cn