Bounded flows in semialgebraic optimization
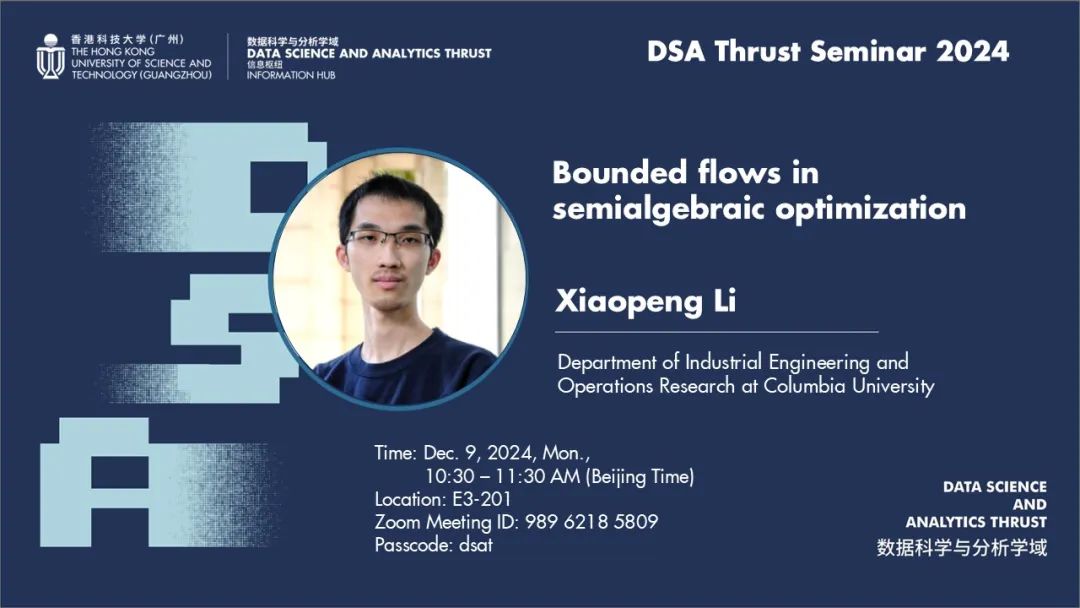
摘要
Classical optimization theory often fails to explain the success of simple algorithms in data science and artificial intelligence due to its heavy reliance on assumptions (e.g., convexity, smoothness and coercivity) that are rarely satisfied or difficult to verify in practice. We seek to establish a new framework that can relax those assumptions or replace them with easily checkable ones, through innovative applications of tools from dynamical systems, semialgebraic geometry, and variational analysis. One of our main findings is that a broad class of optimization problems have bounded gradient flows, which is invaluable for landscape analysis, gradient dynamics, and first-order methods. In this talk, I will discuss both theoretical implications and computational advantages of this line of research, using examples and problems in data science and artificial intelligence such as matrix sensing and neural networks.
演讲者简介
Xiaopeng LI
Columbia University
Xiaopeng Li is currently a fifth-year PhD candidate in the Department of Industrial Engineering and Operations Research at Columbia University, under the supervision of Prof. Cedric Josz. His research focuses on solving nonconvex nonsmooth optimization problems with first-order methods and their applications to data science. His work explores the intersection of variational analysis, dynamical systems and real algebraic geometry. Xiaopeng received his Bachelor’s degree in Mathematics in 2020 from the Chinese University of Hong Kong, Shenzhen.
日期
09 December 2024
时间
10:30:00 - 11:30:00
地点
香港科技大学(广州)E1-2F-201 与 线上
Join Link
Zoom Meeting ID: 989 6218 5809
Passcode: dsat
主办方
数据科学与分析学域
联系邮箱
dsat@hkust-gz.edu.cn