Parsimony Learning from Deep Networks
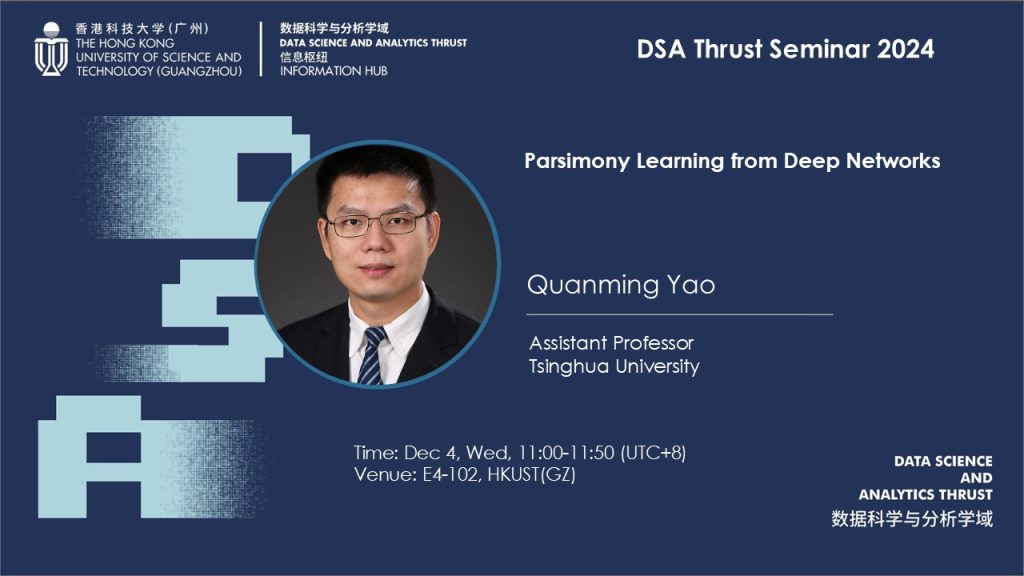
摘要
The scaling law, which involves the brute-force expansion of training datasets and learnable parameters, has become a prevalent strategy for developing more robust learning models. However, due to bottlenecks in data, computation, and trust, the sustainability of the scaling law is a serious concern for the future of deep learning. In this paper, we address this issue by developing next-generation models in a parsimonious manner (i.e., achieving greater potential with simpler models). The key is to drive models using domain-specific knowledge, such as symbols, logic, and formulas, instead of relying on the scaling law. This approach allows us to build a framework that uses this knowledge as “building blocks” to achieve parsimony in model design, training, and interpretation. Empirical results show that our methods surpass those that typically follow the scaling law. We also demonstrate the application of our framework in AI for science, specifically in the problem of drug-drug interaction prediction. We hope our research can foster more diverse technical roadmaps in the era of foundation models.
演讲者简介
Dr. Quanming Yao currently is a tenure-track assistant professor at Department of Electronic Engineering, Tsinghua University. He was a researcher to a senior scientist in 4Paradigm INC, where he set up and led the company's machine learning research team. He obtained his Ph.D. degree at the Department of Computer Science and Engineering of Hong Kong University of Science and Technology (HKUST). He has published 100 top conference and journal papers, with more than 10000 citations. He regularly serves as area chairs for ICML, NeurIPS and ICLR. He is also a receipt of National Youth Talent Plan (China), inaugural winner of Ant Intech Prize, Forbes 30 Under 30 (China), Young Scientist Awards (Hong Kong Institution of Science), and Google Fellowship (in machine learning).
日期
04 December 2024
时间
11:00:00 - 11:50:00
地点
香港科技大学(广州)E4-1F-102
主办方
数据科学与分析学域
联系邮箱
dsarpg@hkust-gz.edu.cn