Generative principal component analysis and fast diffusion sampling
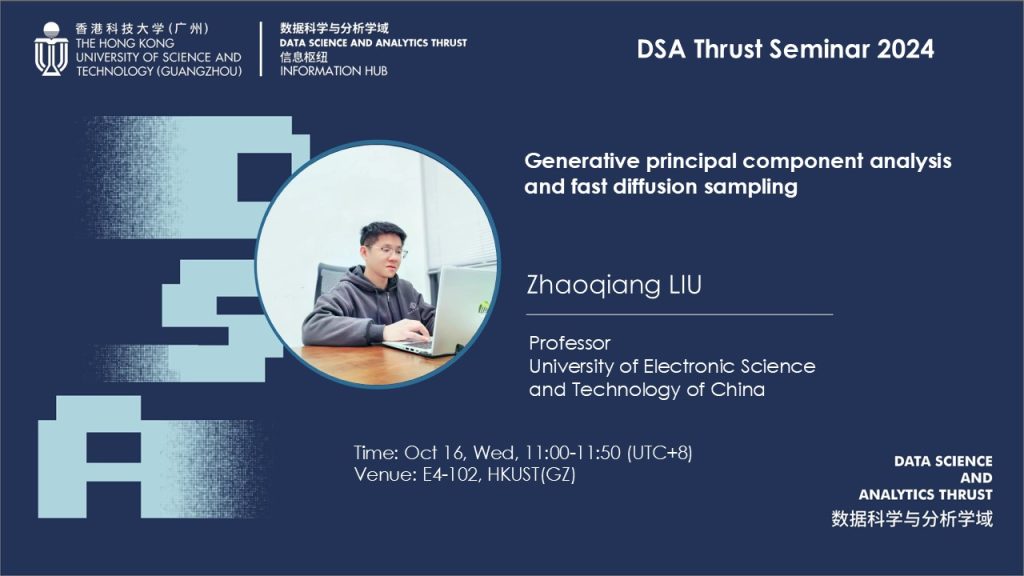
摘要
In this talk, I will first briefly discuss the problem of principal component analysis with generative modeling assumptions. The key assumption is that the underlying signal lies near the range of a Lipschitz continuous generative model with bounded low-dimensional inputs. We propose a quadratic estimator, and show that it enjoys the optimal statistical rate. Moreover, we provide a variant of the classic power method, which projects the calculated data onto the range of the generative model during each iteration. We show that under suitable conditions, this method converges exponentially fast to a point achieving the above-mentioned statistical rate. We perform experiments on various image datasets to illustrate performance gains of our method to the classic power method and the truncated power method devised for sparse principal component analysis.
Next, I will briefly discuss our recent work on accelerating diffusion sampling with optimized time steps. Diffusion (generative) models (DMs) have shown remarkable performance in high-resolution image synthesis, but their sampling efficiency is still to be desired due to the typically large number of sampling steps. Recent advancements in high-order numerical ODE solvers for DMs have enabled the generation of high-quality images with much fewer sampling steps, but most sampling methods simply employ uniform time steps. To address this issue, we propose a general framework for designing an optimization problem that seeks more appropriate time steps for a specific numerical ODE solver for DMs. This optimization problem aims to minimize the distance between the ground-truth solution to the ODE and an approximate solution corresponding to the numerical solver. It can be efficiently solved using the constrained trust region method, taking less than 15 seconds. Our extensive experiments on both unconditional and conditional sampling using pixel- and latent-space DMs validate the effectiveness of the optimized time steps.
演讲者简介
Zhaoqiang Liu is a professor at the School of Computer Science and Engineering, University of Electronic Science and Technology of China. Previously, he was a research fellow in the Department of Computer Science at the National University of Singapore (NUS) and an AI researcher at Huawei Noah's Ark Lab in Hong Kong. He earned his Ph.D. in Mathematics from NUS and he completed his B.Sc. in Mathematics at Tsinghua University. Currently, his main research interests include diffusion models, high-dimensional inverse problems, large language models, and deep reinforcement learning.
日期
16 October 2024
时间
11:00:00 - 11:50:00
地点
香港科技大学(广州)E4-1F-102
主办方
数据科学与分析学域
联系邮箱
dsarpg@hkust-gz.edu.cn