Wiener Graph Deconvolutional Network Improves Graph Self-Supervised Learning
摘要
Graph self-supervised learning (SSL) has been vastly employed to learn representations from unlabeled graphs. Existing methods can be roughly divided into predictive learning and contrastive learning, where the latter one attracts more research attention with better empirical performance. We argue that, however, predictive models weaponed with powerful decoder could achieve comparable or even better representation power than contrastive models. In this work, we propose a Wiener Graph Deconvolutional Network (WGDN), an augmentation-adaptive decoder empowered by graph wiener filter to perform information reconstruction. Theoretical analysis proves the superior reconstruction ability of graph wiener filter. Extensive experimental results on various datasets demonstrate the effectiveness of our approach.
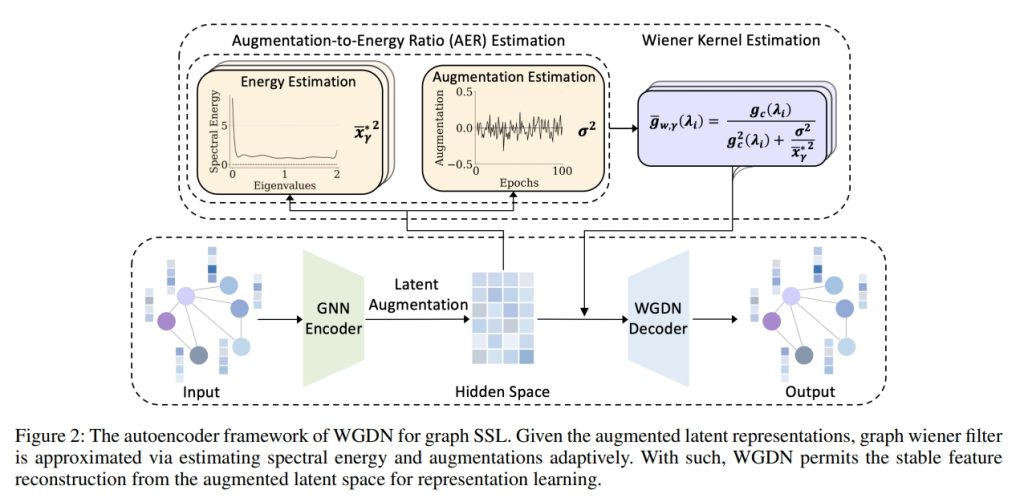
出版文章
Wiener Graph Deconvolutional Network Improves Graph Self-Supervised Learning. Jiashun Cheng, Man Li, Jia Li, and Fugee Tsung.
项目周期
2023
研究领域
Data-driven AI
关键词
ML: Graph-based Machine Learning