Structural Contrastive Pretraining for Cross-Lingual Comprehension
摘要
To present, multilingual language models trained using various pre-training tasks like mask language modeling (MLM) have yielded encouraging results on a wide range of downstream tasks. Despite the promising performances, structural knowledge in cross-lingual corpus is less explored in current works, leading to the semantic misalignment. In this paper, we propose a new pre-training task named Structural Contrast Pretraining (SCP) to align the structural words in a parallel sentence, enhancing the models’ ability to comprehend cross-lingual representations. Concretely, each structural word in source and target languages is regarded as a positive pair in SCP. Since contrastive learning compares positive and negative pairs, an increase in the frequency of negative pairings could enhance the performance of the resulting model. Therefore, we further propose Cross-lingual Momentum Contrast (CL-MoCo) to increase the number of negative pairs by maintaining a large size of the queue. CL-MoCo extends the original Moco approach into cross-lingual training and jointly optimizes the source-to-target language and target-to-source language representations, resulting in a more suitable encoder for cross-lingual transfer. We conduct extensive experiments to validate the proposed approach on three cross-lingual tasks across five datasets such as MLQA, WikiAnn, etc, and results prove the effectiveness of our method.
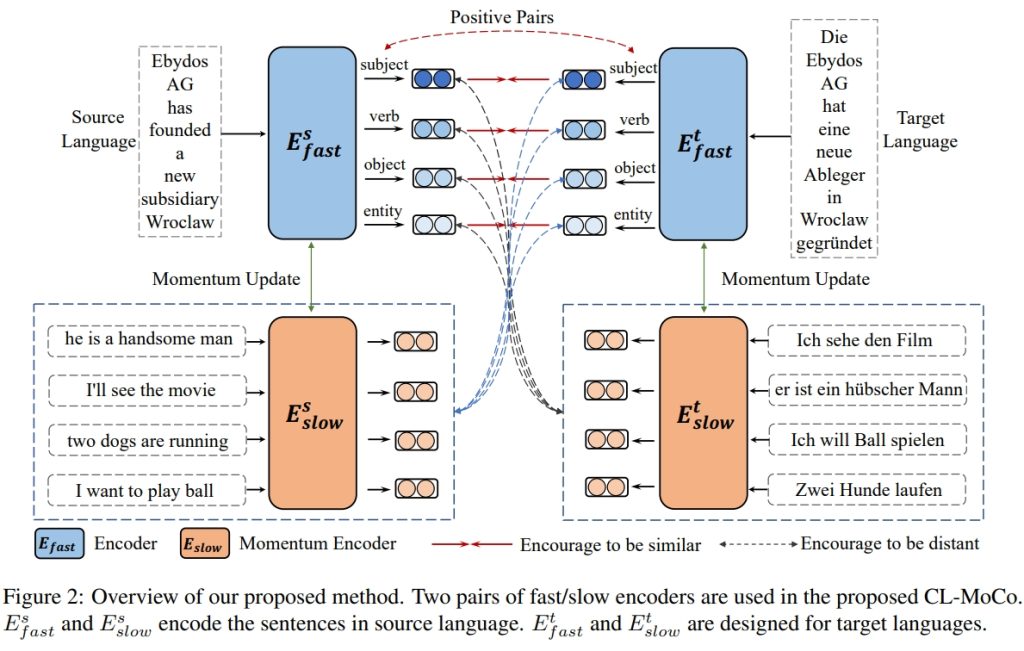
项目成员
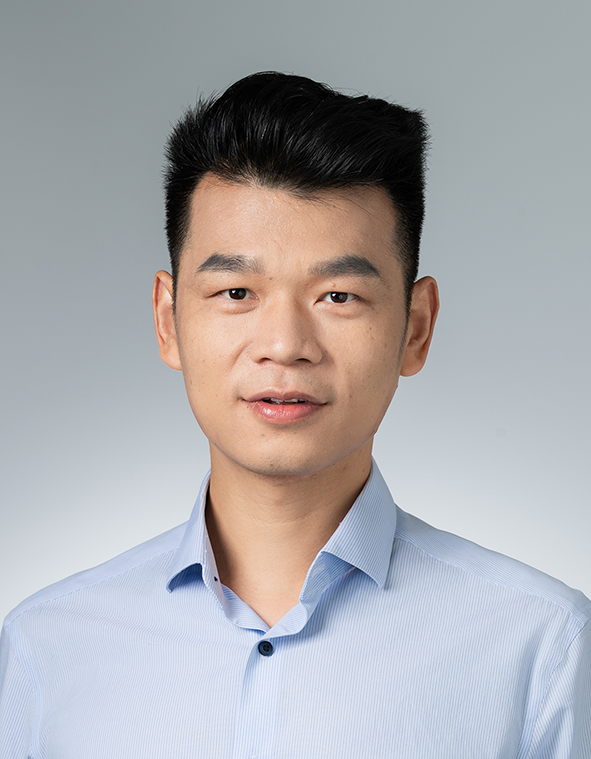
李佳
助理教授
出版文章
Structural Contrastive Pretraining for Cross-Lingual Comprehension. Nuo Chen, Linjun Shou, Tengtao Song, Ming Gong, Jian Pei, Jianhui Chang, Daxin Jiang, and Jia Li.
项目周期
2023
研究领域
Data-driven AI
关键词
Structural Contrast Pretraining