Sobolev Calibration of Imperfect Computer Models
摘要
Calibration refers to the statistical estimation of unknown model parameters in computer experiments, such that computer experiments can match underlying physical systems. This work develops a new calibration method for imperfect computer models, Sobolev calibration, which can rule out calibration parameters that generate overfitting calibrated functions. We prove that the Sobolev calibration enjoys desired theoretical properties including fast convergence rate, asymptotic normality and semiparametric efficiency. We also demonstrate an interesting property that the Sobolev calibration can bridge the gap between two influential methods: L2 calibration and Kennedy and O’Hagan’s calibration. In addition to exploring the deterministic physical experiments, we theoretically justify that our method can transfer to the case when the physical process is indeed a Gaussian process, which follows the original idea of Kennedy and O’Hagan’s. Numerical simulations as well as a real-world example illustrate the competitive performance of the proposed method
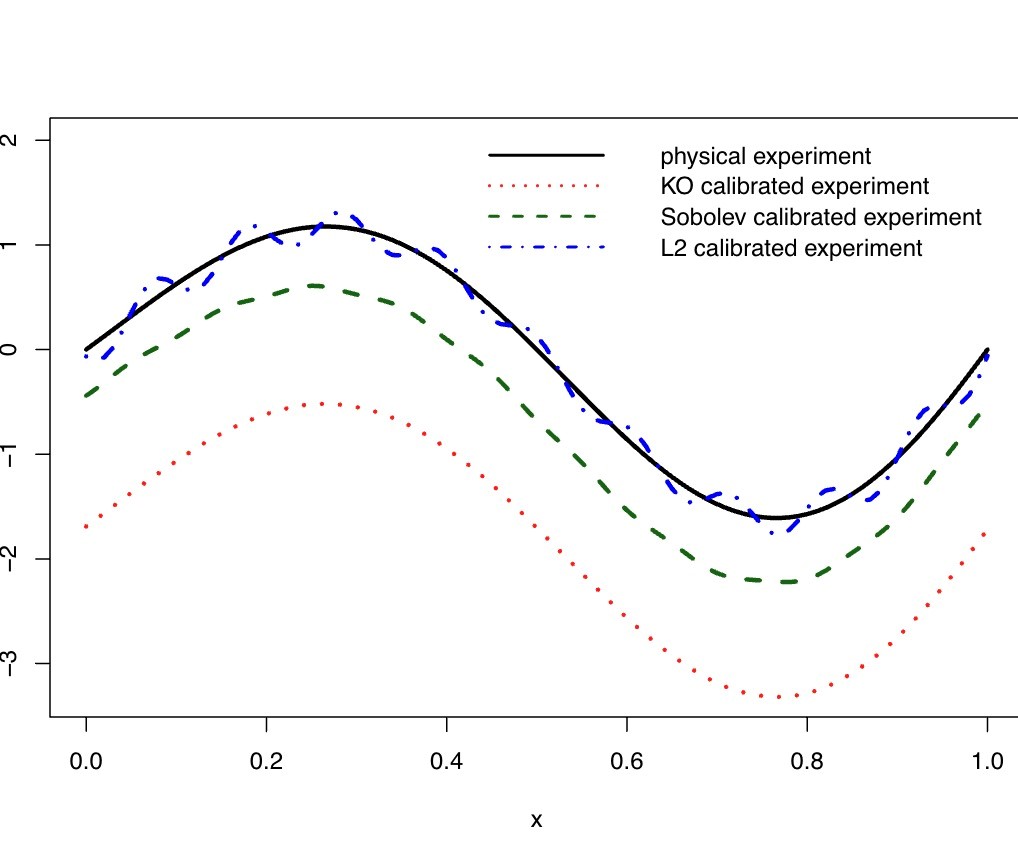
项目成员
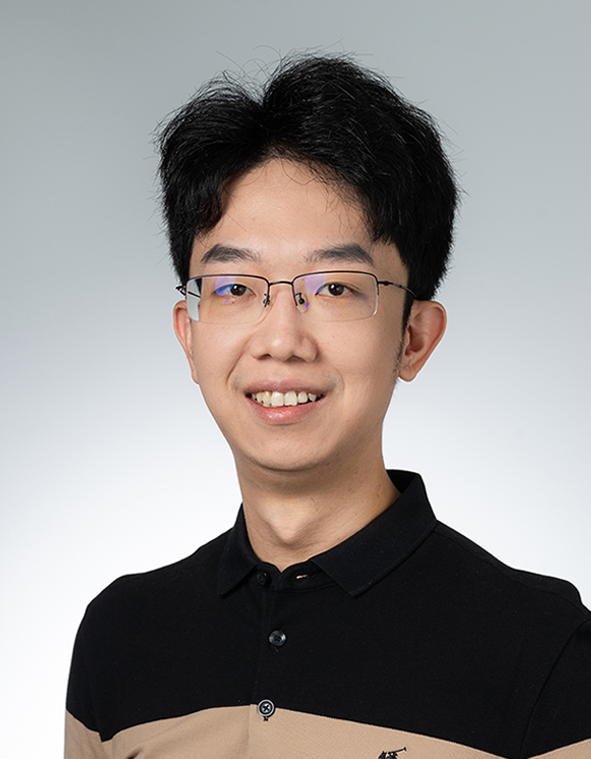
王文佳
助理教授
出版文章
Sobolev Calibration of Imperfect Computer Models. Wenjia Wang, and Qingwen Zhang. Journal of the American Statistical Association.
项目周期
2024
研究领域
统计学习和建模
关键词
Computer experiments, Gaussian process, semiparametric efficiency, uncertainty quantification