Semi-Supervised Hierarchical Graph Classification
摘要
Node classification and graph classification are two graph learning problems that predict the class label of a node and the class label of a graph respectively. A node of a graph usually represents a real-world entity, e.g., a user in a social network, or a document in a document citation network. In this work, we consider a more challenging but practically useful setting, in which a node itself is a graph instance. This leads to a hierarchical graph perspective which arises in many domains such as social network, biological network and document collection. We study the node classification problem in the hierarchical graph where a “node” is a graph instance. As labels are usually limited, we design a novel semi-supervised solution named SEAL-CI. SEAL-CI adopts an iterative framework that takes turns to update two modules, one working at the graph instance level and the other at the hierarchical graph level. To enforce a consistency among different levels of hierarchical graph, we propose the Hierarchical Graph Mutual Information (HGMI) and further present a way to compute HGMI with theoretical guarantee. We demonstrate the effectiveness of this hierarchical graph modeling and the proposed SEAL-CI method on text and social network data.
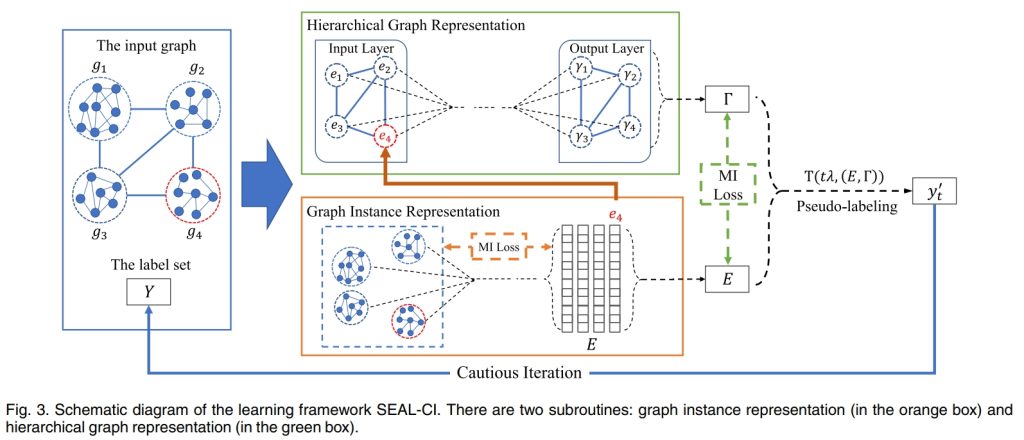
项目成员
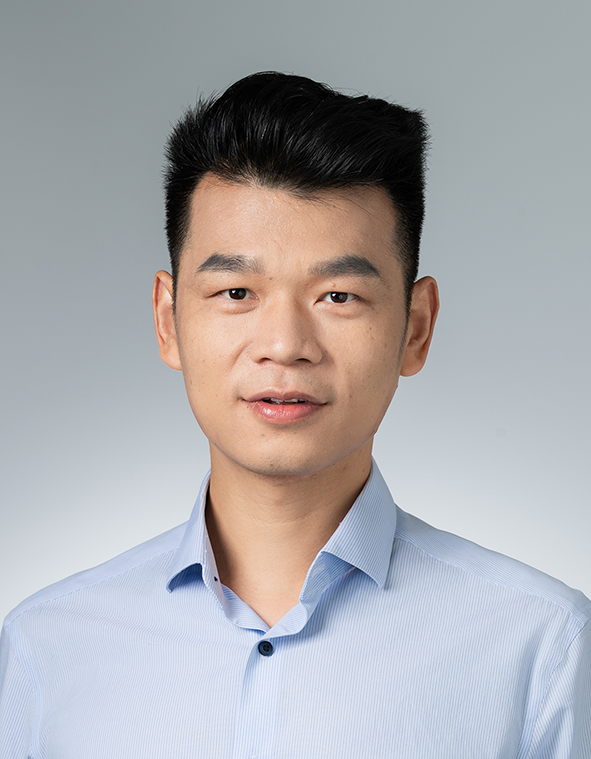
李佳
助理教授
出版文章
Semi-Supervised Hierarchical Graph Classification. Jia Li, Yongfeng Huang, Heng Chang, and Yu Rong.
项目周期
2023
研究领域
Data-driven AI
关键词
graph mutual information, graph representation, Hierarchical graph, Semi-supervised Learning