Alleviating Over-smoothing for Unsupervised Sentence Representation
摘要
Currently, learning better unsupervised sentence representations is the pursuit of many natural language processing communities. Lots of approaches based on pre-trained language models (PLMs) and contrastive learning have achieved promising results on this task. Experimentally, we observe that the over-smoothing problem reduces the capacity of these powerful PLMs, leading to sub-optimal sentence representations. In this paper, we present a Simple method named Self-Contrastive Learning (SSCL) to alleviate this issue, which samples negatives from PLMs intermediate layers, improving the quality of the sentence representation. Our proposed method is quite simple and can be easily extended to various state-of-the-art models for performance boosting, which can be seen as a plug-and-play contrastive framework for learning unsupervised sentence representation. Extensive results prove that SSCL brings the superior performance improvements of different strong baselines (e.g., BERT and SimCSE) on Semantic Textual Similarity and Transfer datasets.
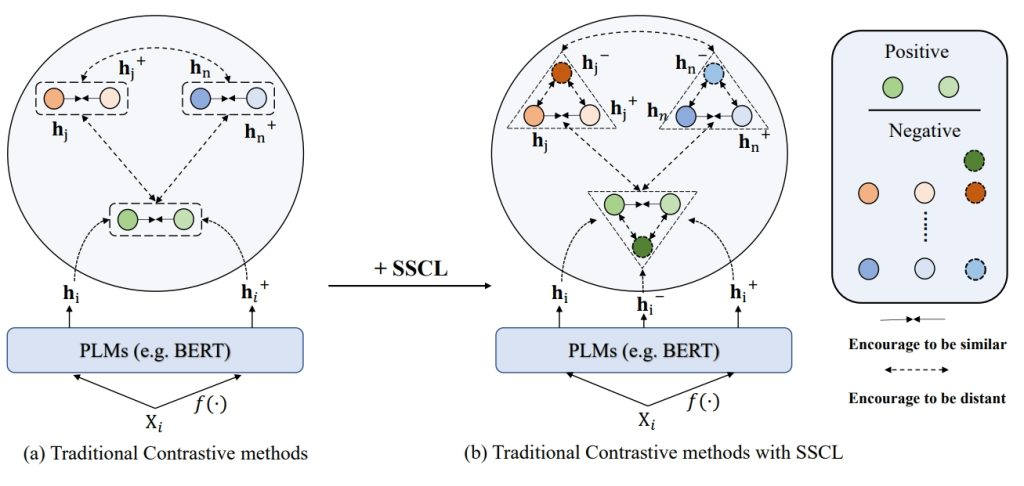
项目成员
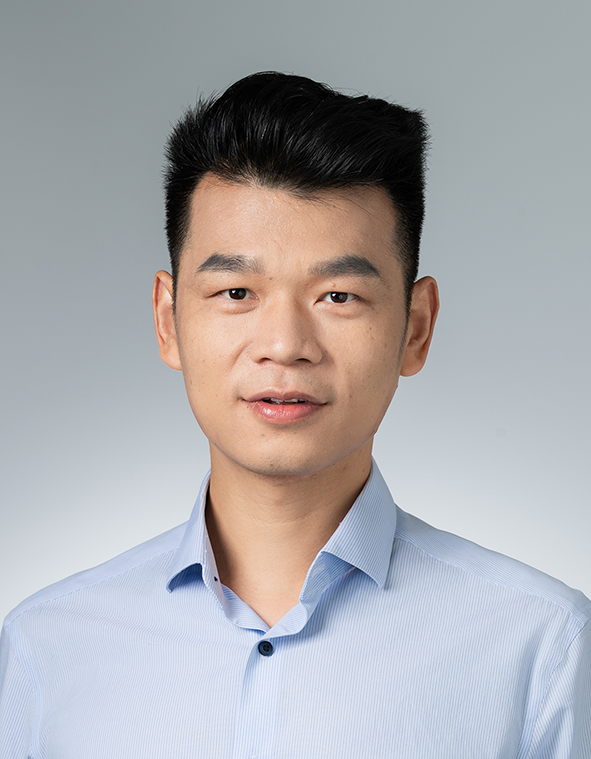
李佳
助理教授
出版文章
Alleviating Over-smoothing for Unsupervised Sentence Representation. Nuo Chen, Linjun Shou, Jian Pei, Ming Gong, Bowen Cao, Jianhui Chang, Jia Li, and Daxin Jiang.
项目周期
2023
研究领域
Data-driven AI
关键词
Pre-trained Language Models, Self-Contrastive Learning