A Plot is Worth a Thousand Words: Model Information Stealing Attacks via Scientific Plots
摘要
Building advanced machine learning (ML) models requires expert knowledge and many trials to discover the best architecture and hyperparameter settings. Previous work demonstrates that model information can be leveraged to assist other attacks, such as membership inference, generating adversarial examples. Therefore, such information, e.g., hyperparameters, should be kept confidential. It is well known that an adversary can leverage a target ML model's output to steal the model's information. In this paper, we discover a new side channel for model information stealing attacks, i.e., models' scientific plots which are extensively used to demonstrate model performance and are easily accessible. Our attack is simple and straightforward. We leverage the shadow model training techniques to generate training data for the attack model which is essentially an image classifier. Extensive evaluation on three benchmark datasets shows that our proposed attack can effectively infer the architecture/hyperparameters of image classifiers based on convolutional neural network (CNN) given the scientific plot generated from it. We also reveal that the attack's success is mainly caused by the shape of the scientific plots, and further demonstrate that the attacks are robust in various scenarios. Given the simplicity and effectiveness of the attack method, our study indicates scientific plots indeed constitute a valid side channel for model information stealing attacks. To mitigate the attacks, we propose several defense mechanisms that can reduce the original attacks' accuracy while maintaining the plot utility. However, such defenses can still be bypassed by adaptive attacks.
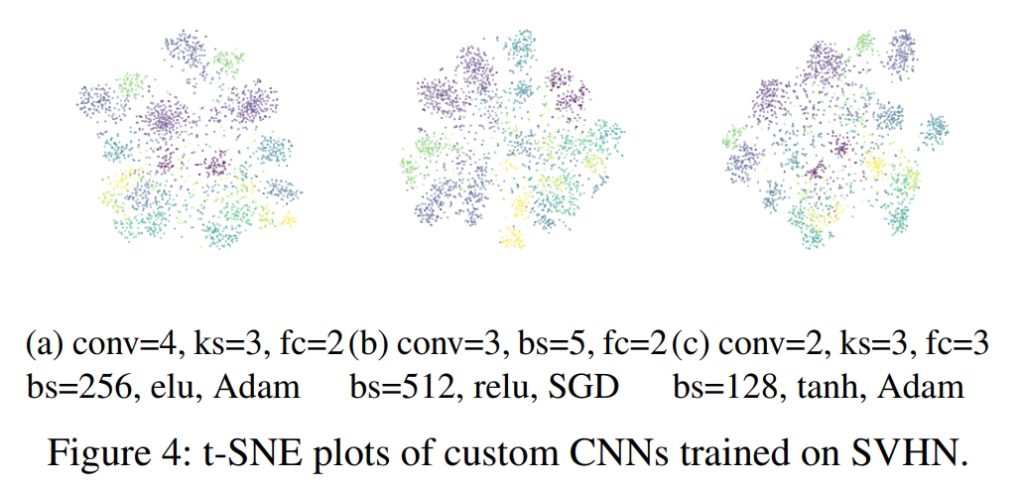
项目成员
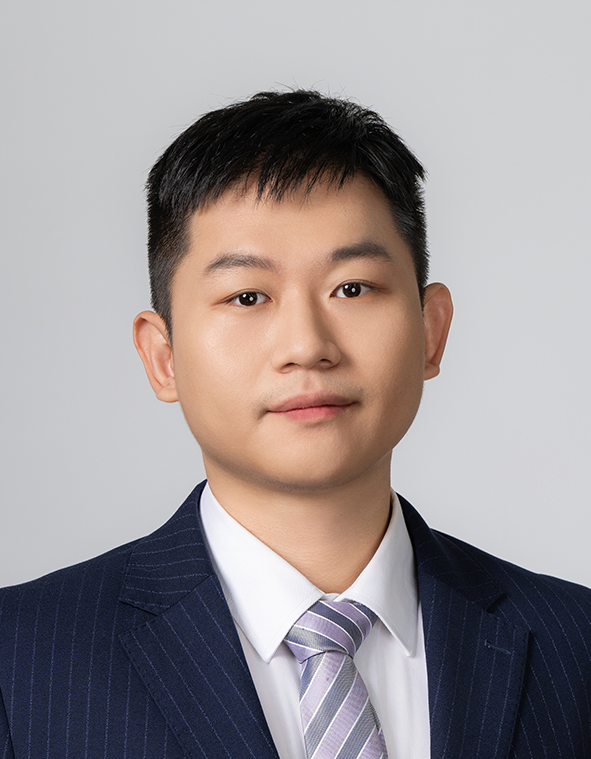
何新磊
助理教授
出版文章
A Plot is Worth a Thousand Words: Model Information Stealing Attacks via Scientific Plots. Boyang Zhang, Xinlei He, Yun Shen, Tianhao Wang, and Yang Zhang.
项目周期
2023
研究领域
Data-driven AI & Machine Learning、Security and Privacy
关键词
Hyperparameter Stealing, t-SNE