Joint Influence: From Theory to Applications
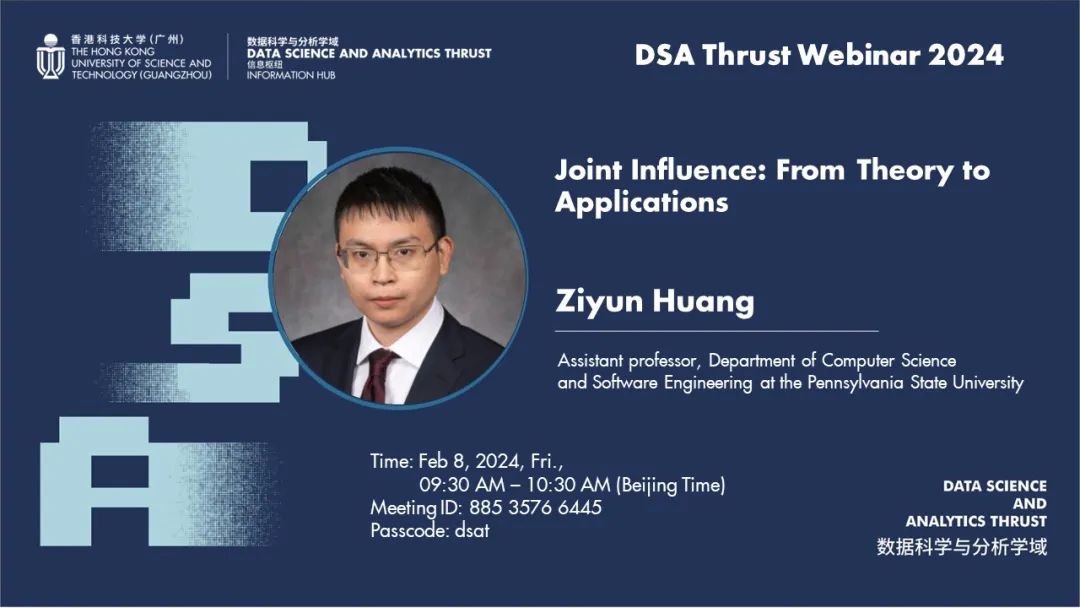
ABSTRACT
Joint influence is a phenomenon that can be commonly observed in many fields. It refers to the scenario where the behavior of one object is jointly determined by a number of other objects. For example, in physics, a particle receives forces from a number of other particles, and such forces can jointly affect the motion of the particle. This phenomenon also arises in areas like social networks, where a set of nodes in a community may have a joint influence on a newly added node. In this presentation, I will introduce a theory on joint influence I have been developing since my doctoral research and show how it can be used to design efficient algorithms for related applications. Particularly, I will show how the joint influence phenomenon can be modeled mathematically in geometric spaces. This leads to several general techniques for joint influence in various settings. Several applications of this theory, including problems that arise in high dimensional computational geometry, data analysis, wireless network, and deep learning, will also be discussed.
SPEAKER BIO
Ziyun HUANG
Assistant professor
the Pennsylvania State University
Dr. Ziyun Huang received his BS degree in Mathematics from the Sun Yat-sen (Zhongshan) University (Guangzhou, China) in 2011 and his PhD degree in Computer Science and Engineering from the State University of New York at Buffalo (Buffalo, USA) in 2018. Following this, he joined the Department of Computer Science and Software Engineering at the Pennsylvania State University, Erie Campus, where he currently holds the position of assistant professor. His research interest lies in the areas of algorithms, computational geometry and their applications in machine learning, big data, and networking. He has published over 25 research papers, many of which appeared in top conferences and journals in theoretical computer science and machine learning. He has designed state-of-the-art algorithms for several fundamental problems. This includes the first extensions of several important geometric problems from low dimensions to very high dimensional space and resolving a long-standing open problem in geometric multi-covering. Dr. Huang is among the pioneering researchers to develop a unified theory of joint influence and to explore the application of this theory to machine learning through the geometric interpretation of deep neural networks.
日期
08 February 2024
时间
09:30:00 - 10:30:00
地点
线上
Join Link
Zoom Meeting ID: 85 3576 6445
Passcode: dsat
主办方
数据科学与分析学域
联系邮箱
dsat@hkust-gz.edu.cn