AI Incubation: Facilitating Human-AI Mutual Learning in Manufacturing Industrial Internet
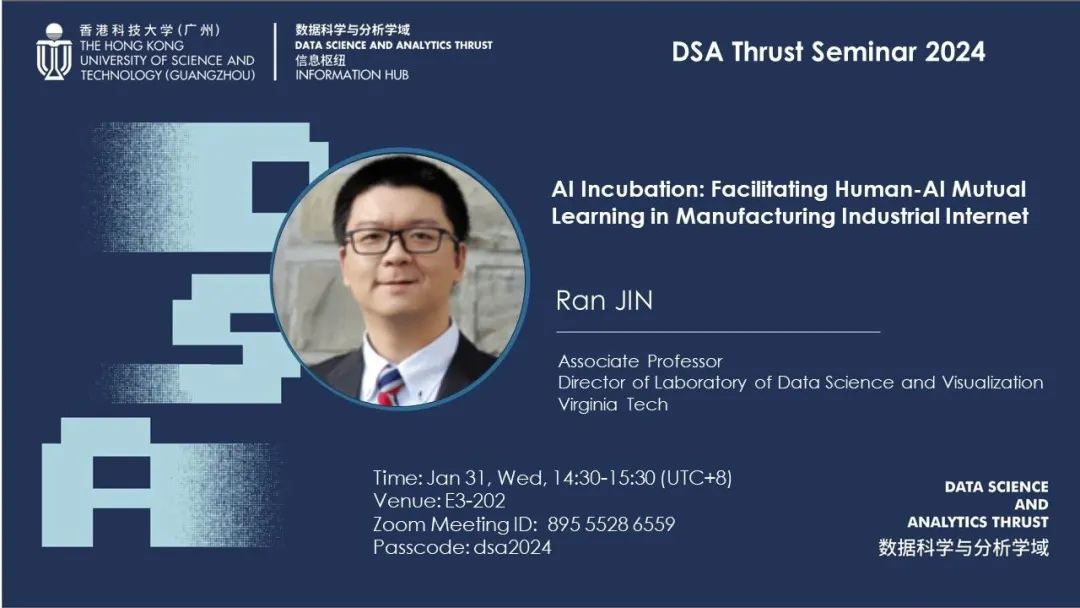
摘要
Both artificial intelligence (AI) and domain knowledge from human experts play an important role in manufacturing decision-making. While smart manufacturing emphasizes a fully automated data-driven decision making, the AI incubation process involves human experts to enhance AI systems by integrating domain knowledge for modeling, data collection and annotation, feature extraction, etc.. Such an AI incubation process will not only enhance the domain knowledge discovery, but also improve the interpretability and trustworthiness of AI methods.
In this talk, I will focus on the knowledge transfer from human experts to a supervised learning problem by learning domain knowledge as interpretable features and rules, which can be used to construct rule-based systems to support manufacturing decision-making, such as process modeling, quality inspection, and anomaly detection. Although many advanced statistical and machine learning methods have shown promising modeling accuracy and efficiency, rule-based systems are still highly preferred and widely adopted due to their interpretability for engineers to comprehend. However, most of the existing rule-based systems are constructed based on deterministic human-crafted rules and inaccurate parameters, e.g., thresholds of decision rules, are suboptimal. On the other hand, the machine learning methods, such as tree models or neural networks, can learn a decision-rule based structure without much interpretation or agreement with domain knowledge. Therefore, the traditional machine learning models and engineers’ domain knowledge cannot be directly improved by learning from data. In this research, we propose an interpretable neural network (INN) model with a center-adjustable Sigmoid activation function to efficiently optimize the rule-based systems. Using the rule-based system from domain knowledge to regulate the INN architecture will not only improve the prediction accuracy with optimized parameters, but also ensure the interpretability by adopting the interpretable rule-based systems from domain knowledge. The proposed INN will be effective for supervised learning problems when rule-based systems are available. The merits of INN model are demonstrated via a simulation study and a real case study in the quality modeling of a semiconductor manufacturing process.
During the seminar, I will also introduce other prior published research results in manufacturing Human-AI collaboration, computation services, and data fusion in Manufacturing Industrial Internet.
演讲者简介
Ran JIN
副教授
Virginia Tech
Dr. Ran Jin is an Associate Professor and the Director of Laboratory of Data Science and Visualization at the Grado Department of Industrial and Systems Engineering at Virginia Tech. He received his Ph.D. degree in Industrial Engineering from Georgia Tech, Atlanta, his Master’s degrees in Industrial Engineering and in Statistics, both from the University of Michigan, Ann Arbor, and his bachelor’s degree in Electronic Engineering from Tsinghua University, Beijing.
Dr. Jin’s research focuses on computation services in Manufacturing Industrial Internet. His research includes data analytics in Manufacturing Industrial Internet, data fusion modeling and decision making, interface between data quality and data-driven modeling, cognitive visualization system design, and interpretable statistical models. He published over 50 journal papers in this area. His research has been broadly used by many manufacturing companies in additive manufacturing, semiconductor manufacturing, and optical fiber manufacturing industries.
Dr. Jin served as President of Engineering Faculty Organization at Virginia Tech, Operations Officer of Faculty Senate, and Co-Chair of Commission on Research at Virginia Tech. He also served as Chair of Quality, Statistics, and Reliability subdivision in INFORMS and Associate Editor for IISE Transactions (Design and Manufacturing). Dr. Jin is currently serving as an Associate Editor for ASME Journal of Manufacturing Science and Engineering, Associate Editor for IEEE Transactions on Automation Science and Engineering, and Associate Editor for INFORMS Journal on Data Science. For more information about Dr. Jin, please visit his faculty website at Virginia Tech: https://ise.vt.edu/ran-jin.
日期
2024年1月31日
时间
14:30:00 - 15:30:00
地点
香港科技大学(广州)E3-2楼-202室
Join Link
Zoom Meeting ID: 895 5528 6559
Passcode: dsa2024
主办方
数据科学与分析学域
联系邮箱
dsarpg@hkust-gz.edu.cn