Knowledge Distillation: Towards Efficient and Compact Neural Networks
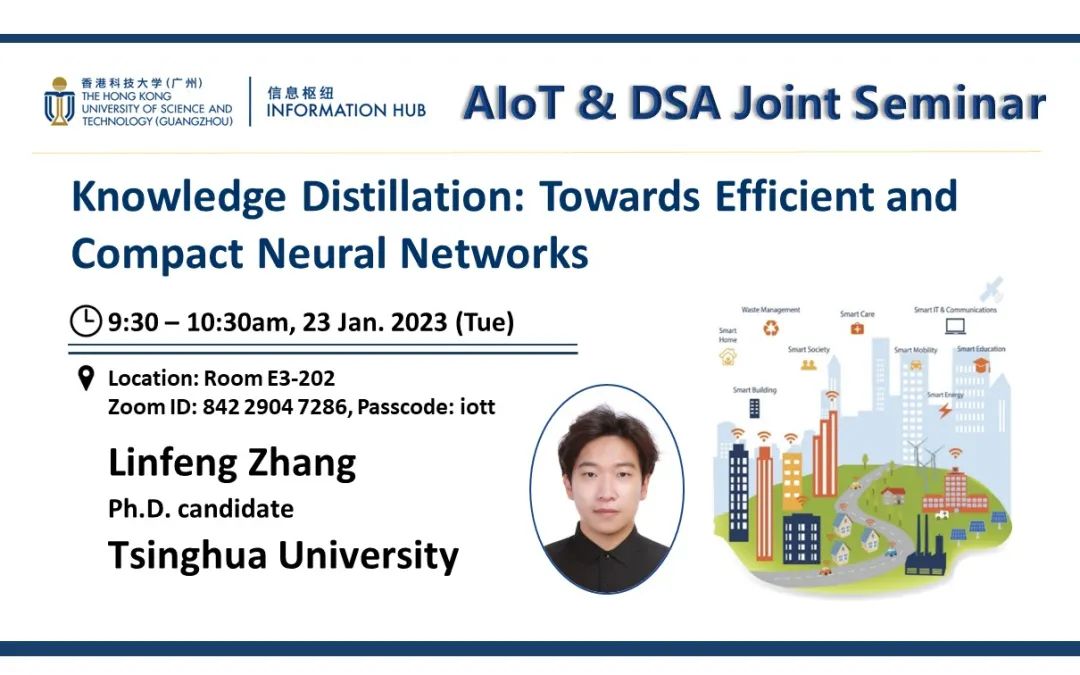
摘要
Deep neural networks usually have a large number of parameters, which makes them powerful in representation learning, but also limits their deployment in real-world application. Knowledge distillation, which aims to transfer the knowledge from an over-parameterized teacher model to an efficient student model, has become one of the most popular method for neural network compression and acceleration. In this report, I will introduce my works on knowledge distillation in the last five years, which mainly focus on two aspects: the fundamental problems in knowledge distillation, and how to apply knowledge distillation to more challenging tasks. Besides, I will introduce the challenge and opportunities of AI model compression in the decade of large models.
演讲者简介
Linfeng ZHANG
Tsinghua University
Linfeng Zhang is a Ph.D. candidate in Tsinghua University. Before that, he obtained his bachelor degree in Northeastern University. Linfeng has been awarded with Microsoft Fellowship in 2020 to encourage his work in knowledge distillation and AI model compression. He has published 13 papers in top-tier conference and journals as the first author. Besides, his research results in AI model compression have been used in many companies such as Huawei, Kwai, DIDI, Polar Bear Technology, Intel and so on.
日期
23 January 2024
时间
09:30:00 - 10:30:00
地点
香港科技大学(广州)E3-2F-202
Join Link
Zoom Meeting ID: 842 2904 7286
Passcode: iott
主办方
数据科学与分析学域 Internet of Things Thrust
联系邮箱
dsat@hkust-gz.edu.cn iott@hkust-gz.edu.cn