Out-of-Distribution Generalized Graph Machine Learning
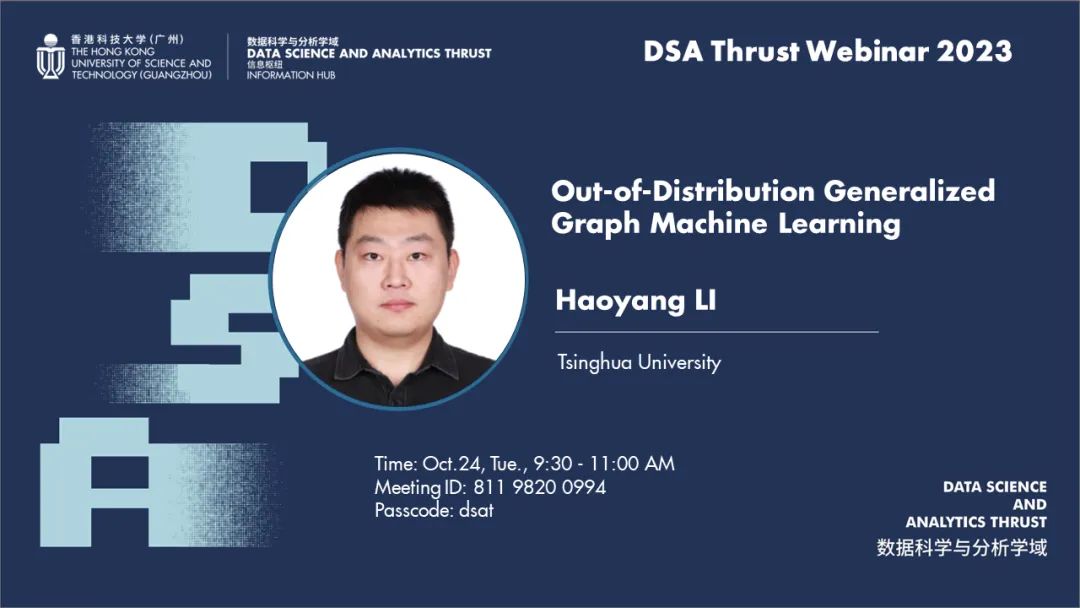
ABSTRACT
Graphs are widely used to model the complex relationships and dependencies between entities in diverse datasets, including online social networks, transportation networks, molecule structures, protein-protein interactions, etc. Graph machine learning, especially graph neural networks, has achieved great success in both academia and industry, primarily due to the powerful representation learning capability on graph-structured data. The majority of current graph machine learning models rely on the in-distribution hypothesis, which posits that testing and training graph data are sampled from the identical distribution. However, such a hypothesis is difficult to be satisfied in the real world. There will inevitably be scenarios with distribution shifts between testing and training environments due to the uncontrollable underlying data generation mechanism. Because of lacking the ability of out-of-distribution generalization, these methods fail dramatically with significant performance drops under distribution shifts. This talk delves into research aimed at addressing this challenge by developing fundamental innovative graph machine learning models with strong out-of-distribution generalization ability. I will deeply discuss the strategies for ensuring graph machine learning models get rid of spurious correlations but capture invariances, achieving reliable and safe generalization to in-the-wild testing data, and think about some potential applications.
SPEAKER BIO
Haoyang LI
Tsinghua University
Dr. Haoyang Li received his Ph.D. in computer science and technology from Tsinghua University in 2023. His research interests focus on the generalization capability of graph machine learning models and its interdisciplinary applications. He has published several high-quality papers at top AI/ML conferences and in journals, including Neural Information Processing Systems (NeurIPS), International Conference on Learning Representations (ICLR), Knowledge Discovery and Data Mining (KDD), International Joint Conference on Artificial Intelligence (IJCAI), and IEEE Transactions on Knowledge and Data Engineering (TKDE). He also organized several tutorials at international conferences like WWW 2023 and IJCAI 2023. Dr. Li won the best paper award at ACM MM 2023 workshop on multimedia content generation and evaluation. He led a team of over ten post-graduate students to develop an open-sourced library AutoGL on automatic graph machine learning with over 1,000 Github stars. Additionally, some of his research has been powering several billion-scale applications in Tencent and Alibaba. Dr. Li has received multiple prestigious awards, including the Tsinghua Outstanding Doctoral Dissertation Award, the Beijing Outstanding Graduate Student Award, and the Tencent Rhino-Bird Elite.
日期
24 October 2023
时间
09:30:00 - 11:00:00
地点
线上
Join Link
Zoom Meeting ID: 811 9820 0994
Passcode: dsat
主办方
数据科学与分析学域
联系邮箱
dsat@hkust-gz.edu.cn