Simulating Learning Methodology (SLeM): Theory, Algorithms and Applications
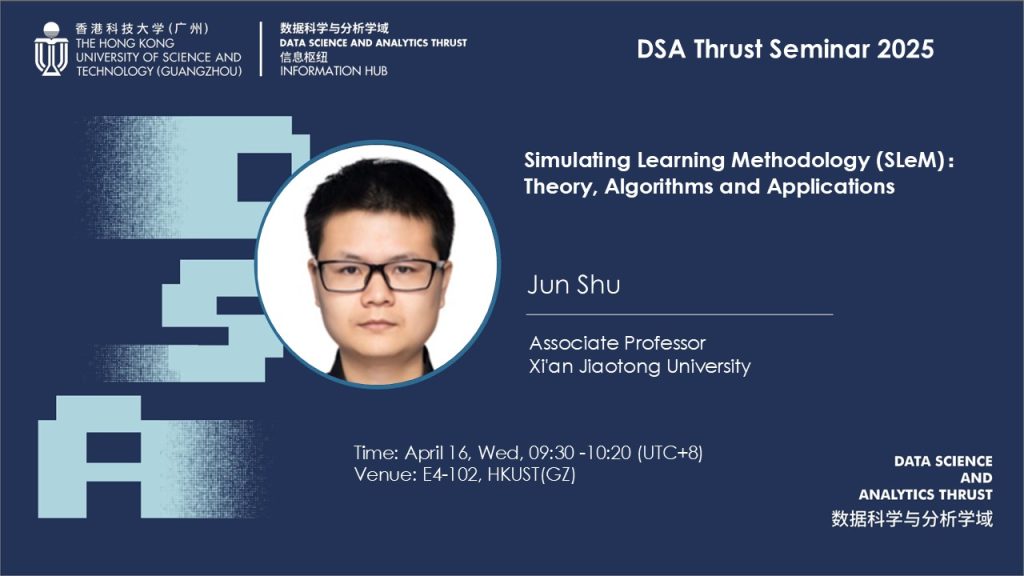
摘要
Recently, large foundation models have made remarkable strides in the field of artificial intelligence. Unlike traditional deep learning models that are carefully crafted for specific tasks, large foundation models exhibit exceptional capabilities in addressing complex challenges such as cross-task generalization—an ability widely attributed to the phenomenon of intelligent emergence. However, a substantial gap remains between the brute-force paradigm underpinning large foundation models and the resource-constrained nature of academic research.
This talk presents the Simulating Learning Methodology (SLeM) framework, which enables task-level machine learning along with theoretical guarantees grounded in statistical learning theory. To demonstrate the effectiveness of the SLeM framework, we introduce a series of machine learning automation algorithms, showcasing its promising applicability to real-world, dynamic problem settings. For more information, please refer to our recent publication, “Simulating Learning Methodology (SLeM): An Approach to Machine Learning Automation,” in National Science Review.
演讲者简介
Jun Shu is an Associate Professor at Xi'an Jiaotong University. His research interests include the fundamental theories and algorithms of machine learning.. He has published over 20 peer-reviewed papers in top-tier venues, including JMLR, TPAMI, NeurIPS, and ICML. His work has received more than 1,600 citations according to Google Scholar. Dr. Shu serves as a regular reviewer for several leading journals and conferences in the field of artificial intelligence and machine learning, including IEEE TPAMI, TMLR, IEEE TIP, NeurIPS, ICML, ICLR, and etc.
日期
16 April 2025
时间
09:30:00 - 10:20:00
地点
E4-102(HKUST-GZ)
联系邮箱
dsarpg@hkust-gz.edu.cn