Large Graphical Models: Estimation and Sampling
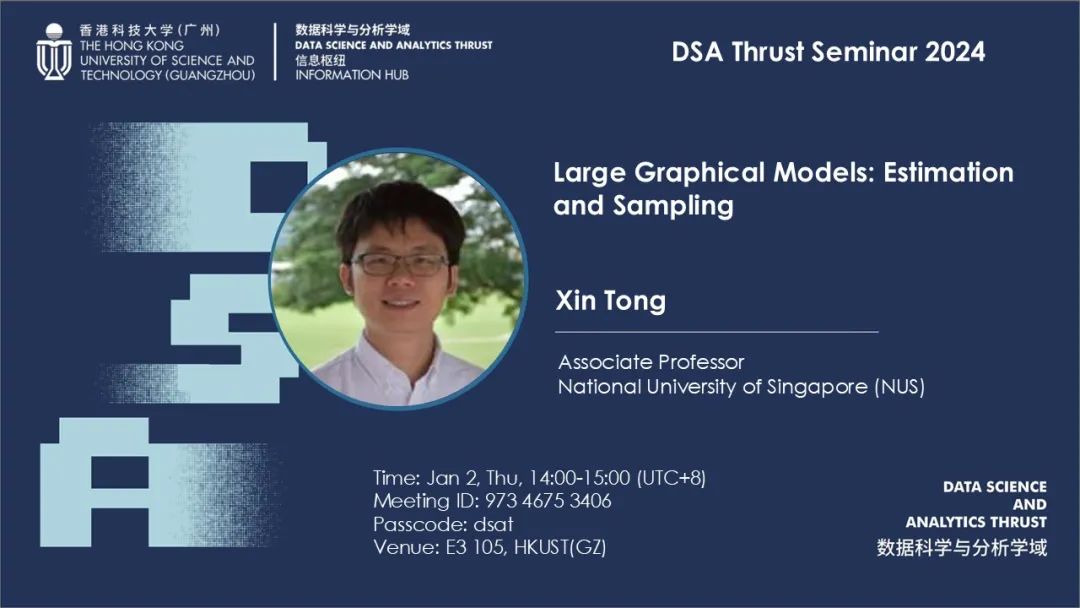
摘要
Distributions on large graphical model often exhibit sparse or incoherent features. Intuitively, such incoherency implies low intrinsic dimensionality, which can be exploited for efficient approximation and computation of complex distributions. Existing approximation theory mainly considers the joint distributions, which does not guarantee that the marginal error is small. In this work, we establish a dimension independent error bound for the marginals of approximate distributions. Such l_infty approximation error is achieved by Stein's method, and we propose a condition that quantifies how a distribution is incoherent. We also show how to obtain uniform error bound given different sparsity conditions that characterize incoherence. The l_inf approximation bound motivates to sparsify existing approximation methods to respect the locality. As examples, we show how to use score matching to avoid the dimension dependence in the approximation error. We also show how to use parallel Gibbs sampling to efficiently accelerate the sampling.
演讲者简介
Xin Tong
副教授
National University of Singapore (NUS)
Xin Tong is an Associate Professor at the National University of Singapore (NUS) , department of mathematics. He received his Ph.D. from Princeton University in 2013. He was a postdoc at the Courant Institute of Mathematical Science and later joined NUS. His works focus on the theoretical aspects of applied mathematics, solving problems in uncertainty quantification, operations research and statistical learning.
日期
02 January 2025
时间
14:00:00 - 15:00:00
地点
香港科技大学(广州)E3-1楼-105室
Join Link
Zoom Meeting ID: 973 4675 3406
Passcode: dsat
主办方
数据科学与分析学域
联系邮箱
dsat@hkust-gz.edu.cn