Kernel Packet: An Exact and Scalable Algorithm for Gaussian Process Regression with Matérn Correlations
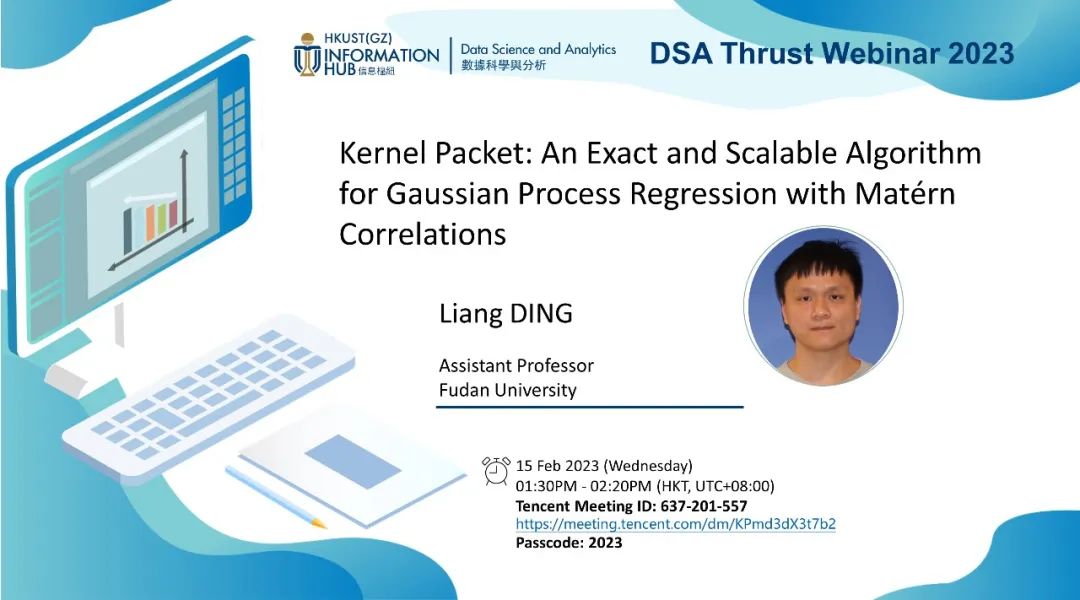
摘要
We develop an exact and scalable algorithm for one-dimensional Gaussian process regression with Matérn correlations whose smoothness parameter ν is a half-integer. The proposed algorithm only requires O(v^3n) operations and O(vn) storage. This leads to a linear-cost solver since ν is chosen to be fixed and usually very small in most applications. The proposed method can be applied to multi-dimensional problems if a full grid or a sparse grid design is used. The proposed method is based on a novel theory for Matérn correlation functions. We find that a suitable rearrangement of these correlation functions can produce a compactly supported function, called a "kernel packet". Using a set of kernel packets as basis functions leads to a sparse representation of the covariance matrix that results in the proposed algorithm. Simulation studies show that the proposed algorithm, when applicable, is significantly superior to the existing alternatives in both the computational time and predictive accuracy.
演讲者简介
Liang DING
助理教授
Fudan University
Liang Ding is an Assistant Professor in the Data Science School at Fudan University. Prior to joining Fudan, he was a postdoctoral fellow in the ISEN department at Texas A&M. He received his Ph.D. degree in IEDA at HKUST. His research interests include Gaussian Processes, kernel methods, and simulation optimization.
日期
15 February 2023
时间
13:30:00 - 14:20:00
地点
香港科技大学(广州)E1-1F-101
Join Link
Tencent Meeting ID:
637-201-557
Passcode: 2023
主办方
数据科学与分析学域
联系邮箱
dsarpg@hkust-gz.edu.cn