Star Flow: Accelerating Online Processing of Astronomic Data on Heterogeneous Processors
摘要
Several new astronomy projects, including the Large Synoptic Survey Telescope (LSST) in the US, and the Ground-based Wide-Angle Cameras (GWAC) in China, are building either a single, large telescope or an array of smaller cameras to continuously observe a considerable portion of the sky. Once in operation, these instruments will produce observation images every few seconds. Furthermore, complex processing is required to catalog raw images online, and transient objects, such as stars that change positions or brightness, should be detected from the data instantly to enable timely follow-up observation. Consequently, accelerating the online processing of these data is crucial to meet the demands of these projects and to facilitate astronomic discoveries.
There have been initial studies on utilizing commodity computer processors such as multicore CPUs (Central Processing Units) and GPUs (Graphics Processing Units) to parallelize computation on astronomical data. However, the pipeline processing of camera data during normal observations is complex, involving image processing, astrometry computation, and database operations. As a result, parallelizing this pipeline remains a challenging open problem, especially in a heterogeneous environment with both multicore CPUs and GPUs. Moreover, recent developments in heterogeneous processors, such as the Intel Phi co-processors for the CPU, and the dynamic scheduling capabilities in NVIDIA Kepler GPUs to involve multiple CPU cores, pose interesting opportunities to parallel computing.
We propose Star Flow, an open-source software project that utilizes state-of-the-art many-core CPUs with their co-processors as well as GPUs to parallelize the processing pipeline of astronomical observation data. In Star Flow, we will tackle three major components in the processing pipeline: (1) source extraction, (2) image subtraction, and (3) cross match. In source extraction, the tabular attributes of hundreds of thousands of stars and galaxies are extracted from each observation image. Image subtraction and cross match are two alternatives of finding transient objects with the difference being whether the input are observation images or tabular data.
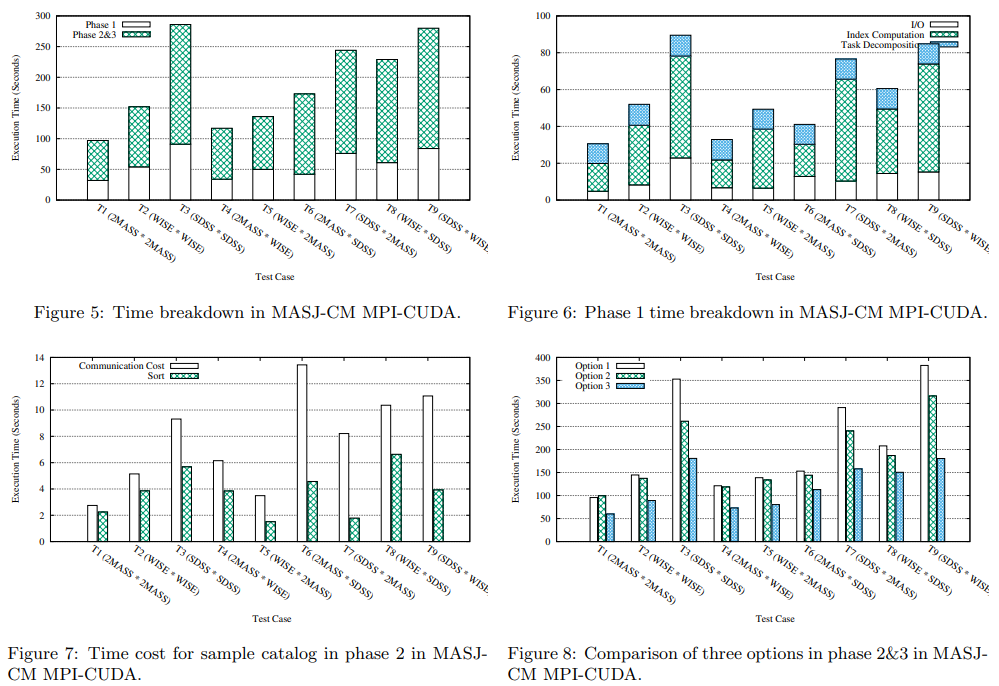
项目成员
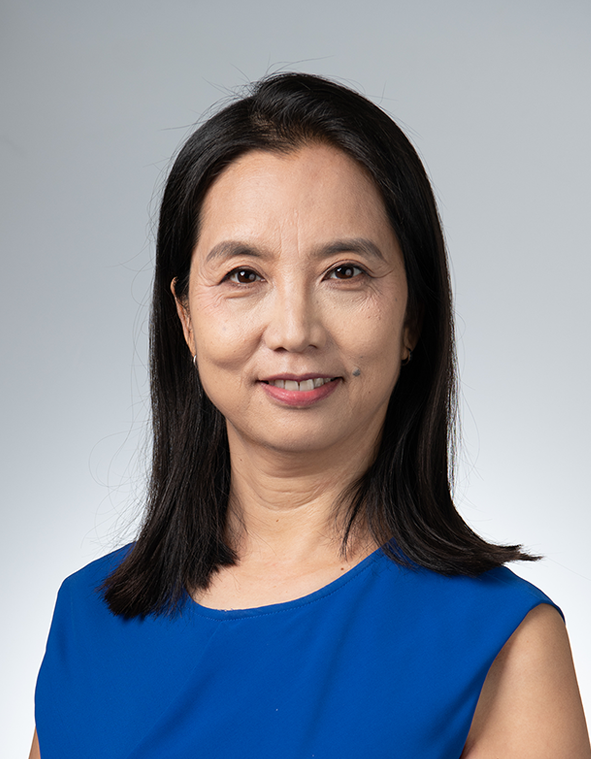
罗琼
教授
出版文章
1. Multi-Assignment Single Joins for Parallel Cross-Match of Astronomic Catalogs on Heterogeneous Clusters. Xiaoying Jia, and Qiong Luo. SSDBM 2016: 12:1-12:12.
2. Cross-Matching Large Astronomical Catalogs on Heterogeneous Clusters. Xiaoying Jia, Qiong Luo, and Dongwei Fan. ICPADS 2015: 617-624.
3. Accelerating astronomical source extraction with graphics processors. Baoxue Zhao. MPhil Thesis, HKUST, Dec 2014.
4. Parallelizing Astronomical Source Extraction on the GPU. Baoxue Zhao, Qiong Luo, and Chao Wu. IEEE 9th International Conference on e-Science, Beijing, China, Oct 2013, pp 88-97.
5. Accelerating Astronomical Image Subtraction on Heterogeneous Processors. Yan Zhao, Qiong Luo, Senhong Wang, and Chao Wu. IEEE 9th International Conference on e-Science, Beijing, China, Oct 2013, pp 70-77.
6. Accelerating In-Memory Cross Match of Astronomical Catalogs. Senhong Wang, Yan Zhao, Qiong Luo, Chao Wu, and Yang Xv. IEEE 9th International Conference on e-Science, Beijing, China, Oct 2013, pp 326-333.
项目周期
2013-Present
研究领域
高性能数据分析系统