Deep Insights into Noisy Pseudo Labeling on Graph Data
摘要
Pseudo labeling (PL) is a wide-applied strategy to enlarge the labeled dataset by self-annotating the potential samples during the training process. Several works have shown that it can improve the graph learning model performance in general. However, we notice that the incorrect labels can be fatal to the graph training process. Inappropriate PL may result in the performance degrading, especially on graph data where the noise can propagate. Surprisingly, the corresponding error is seldom theoretically analyzed in the literature. In this paper, we aim to give deep insights of PL on graph learning models. We first present the error analysis of PL strategy by showing that the error is bounded by the confidence of PL threshold and consistency of multi-view prediction. Then, we theoretically illustrate the effect of PL on convergence property. Based on the analysis, we propose a cautious pseudo labeling methodology in which we pseudo label the samples with highest confidence and multi-view consistency. Finally, extensive experiments demonstrate that the proposed strategy improves graph learning process and outperforms other PL strategies on link prediction and node classification tasks.
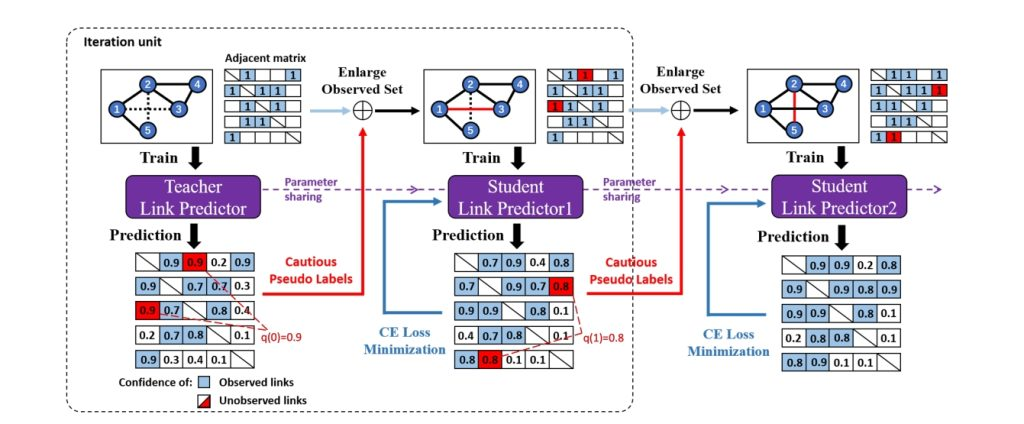
出版文章
Deep Insights into Noisy Pseudo Labeling on Graph Data. Botao WANG, Jia Li, Yang Liu, Jiashun Cheng, Yu Rong, Wenjia Wang, and Fugee Tsung.
项目周期
2023
研究领域
Data-driven AI
关键词
Cautious, Error analysis, Graph data, Pseudo labeling