Data Acquisition
摘要
In many supervised ML projects, the main bottleneck is the lack of sufficient labeled train data (a.k.a. data-centric ML), not which ML models to use and how to optimize these models (a.k.a. model-centric ML), especially for ML practitioners. The process of getting more labeled data is known as data acquisition, which is categorized into two classes: human-in-the-loop and automatic data acquisition. Human-in-the-loop data acquisition includes weak supervision where users need to define rules (e.g., Snorkel, data programming), and crowd- and expert-sourcing. Automatic data acquisition uses automatic methods to obtain more train data.
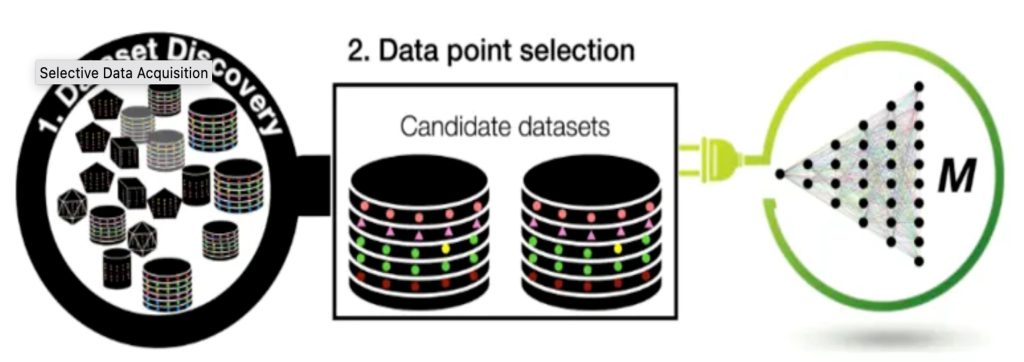
出版文章
1. Selective Data Acquisition in the Wild for Model Charging. Chengliang Chai, Jiabin, Nan Tang, Guoliang Li, and Yuyu Luo.
2. Automatic Data Acquisition for Deep Learning. Jiabin Liu, Fu Zhu, Chengliang Chai, Yuyu Luo, and Nan Tang.
项目周期
2022-Present
研究领域
Data-centric AI
关键词
acquisition, ML, training data