Powers of Function Composition in Deep Neural Networks
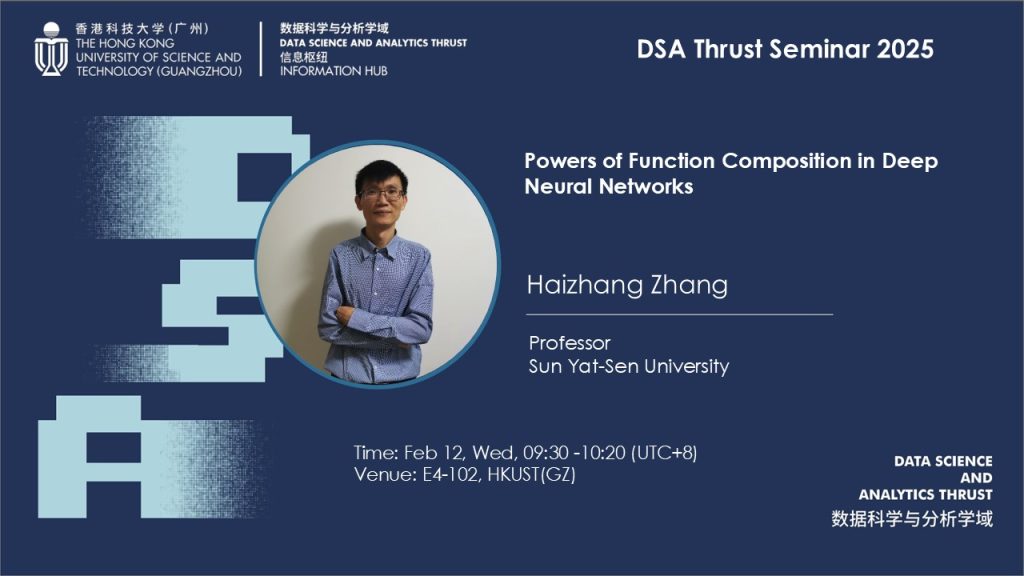
ABSTRACT
It is well-known that major breakthroughs in applied and computational mathematics in the past were majorly stimulated by new function representation systems, which include power series, splines, Fourier series, wavelets, and intrinsic mode functions. The recent example is deep neural networks for machine learning. Unlike those linear representation systems, deep neural networks are nonlinear and the essence is to generate representation functions by composition of the linear functions and an activation function. It is a mystery why function composition is so efficient in representing complex functions. We contribute to understanding the power of function composition in deep neural networks from two perspectives. The first one is to explain why function composition is more inclined to generate complex functions. The second one is to understand the efficiency of function approximation by compositions.
SPEAKER BIO
Haizhang Zhang has been a professor of mathematics at Sun Yat-sen University since June 2010. He received his Ph.D. from Syracuse University, USA in May 2009. From June 2009 to May 2010, he was a postdoctoral research fellow at University of Michigan, Ann Arbor. Prof. Zhang’s research interests include applied and computational harmonic analysis, machine learning, sampling theory, and time–frequency analysis. He has led several significant research projects funded by the National Natural Science Foundation of China and the Guangdong Natural Science Foundation. His contributions include advancements in reproducing kernel Banach spaces and their applications in machine learning.
Date
12 February 2025
Time
09:30:00 - 10:20:00
Location
E4-102, HKUST(GZ)
Event Organizer
Data Science and Analytics Thrust
dsarpg@hkust-gz.edu.cn