Optimal Online Learning under Sequential and Uncertain Competitions
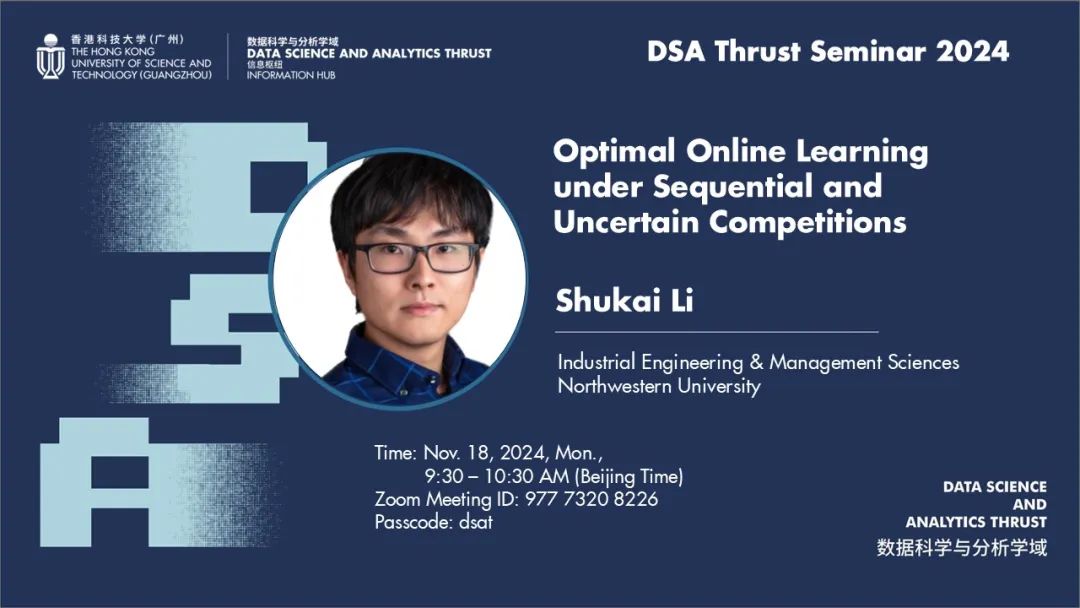
ABSTRACT
Online learning for operations management has been extensively studied in the past decade. However, literature often assumes the decision maker is the only agent in the market, overlooking the role of competition. Particularly, managerial insights derived for single-agent markets can be inaccurate or incorrect for realistic, multi-agent markets. We extend online learning to operations management in competitive settings. The following two questions are investigated: (1) What policies achieve optimal efficiency in uncertain competitions? This question is hard, as proving convergence for multi-agent online learning is an ongoing challenging practice: The first quantitative last-iterate convergence result was only established in 2020 but relied on a restrictive assumption that fails to apply to many fundamental operations management problems. (2) What mechanisms drive agents toward equilibrium or collusion? This issue attracts attention of economists and anti-trust agency concerned about whether algorithmic pricing leads to unfair price increases.
In this talk, I will demonstrate our results with an example of sequential price competition among multiple sellers over a selling horizon of periods. In each period, sellers simultaneously offer their prices and subsequently observe their respective demand that is unobservable to competitors. The realized demand of each seller depends on the prices of all sellers following a private unknown linear model. We propose a least-squares estimation then gradient optimization policy, which does not require sellers to communicate demand information or coordinate price experiments throughout the selling horizon. We show that our policy, when employed by all sellers, leads at a fast convergence rate to the Nash equilibrium prices, which avoids collusion and ensures compliance with anti-trust laws. Meanwhile, each seller achieves optimal efficiency, attaining an optimal order-of- regret relative to a strong dynamic benchmark policy.
SPEAKER BIO
Shukai LI
Northwestern University
Shukai Li is a final-year Ph.D. candidate in Industrial Engineering & Management Sciences at Northwestern University. His research interests are at the intersection of data-driven decision-making, stochastic models, and their applications in healthcare, revenue management, and service operations. Shukai’s work contributes novel methodologies in multi-agent online learning and the non-asymptotic analysis of queues. His research has been published in top-tier journals including Operations Research (1 accepted, 3 major revisions), Mathematics of Operations Research (1 minor revision), and SIAM Journal of Numerical Analysis (1 accepted). Shukai holds dual bachelor’s degrees in medicine and mathematics from Peking University, awarded in 2018.
Date
18 November 2024
Time
09:30:00 - 10:30:00
Location
Online
Join Link
Zoom Meeting ID: 977 7320 8226
Passcode: dsat
Event Organizer
Data Science and Analytics Thrust
dsat@hkust-gz.edu.cn