From Statistical Foundations to the Frontiers of AI
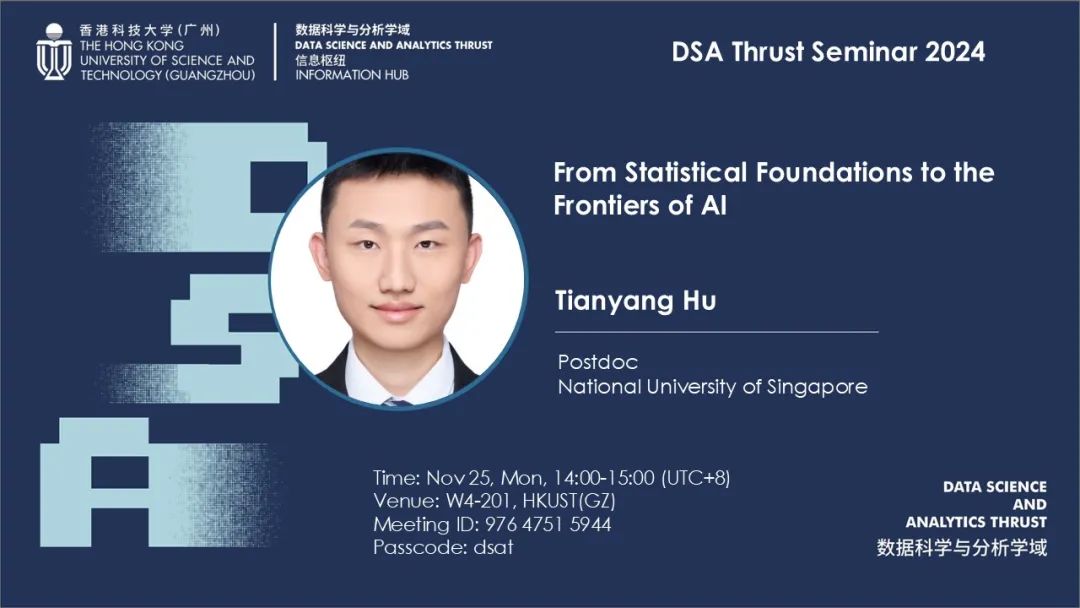
ABSTRACT
The success of artificial intelligence (AI) relies on a seamless integration of theory and practice. This talk will explore how fundamental understandings can drive progress at the frontiers of AI. We begin by establishing the statistical foundation of deep learning, investigating why neural networks are particularly effective for high-dimensional, complex data in traditional discriminative tasks. Our work demonstrates that neural networks can achieve statistically optimal sample complexity and adapt to low-dimensional structures embedded within data. Further, we show how this perspective can be impactful in the era of large language models (LLMs), shedding light on the “scaling of intelligence”. We then delve into the inner workings of networks, examining what models learn from data. By presenting a unified view of feature learning from the perspective of distribution preservation, we highlight a pathway to more effective feature utilization in downstream discriminative and generative tasks. While the relationship between training data and learned model parameters becomes increasingly opaque in the LLM era, there is a promising avenue to explore: the in-context learning abilities of LLMs offer fresh insight into this complex relationship, holding potential as a guiding principle for next-generation LLMs.
SPEAKER BIO
Tianyang HU
National University of Singapore
Dr. Tianyang Hu is a Postdoc at the National University of Singapore, working with Prof. Kenji Kawaguchi. Previously, he was a research scientist in the AI Theory Group of Huawei's Noah's Ark Lab. He earned his PhD in Statistics from Purdue University under the supervision of Prof. Guang Cheng. Dr. Hu's research interests lie in the intersection of Statistics and AI, with a primary focus on statistical deep learning, representation learning, and generative modeling. His work has contributed to the theoretical understanding of deep learning and inspired practical advancements at the frontiers of AI.
Date
25 November 2024
Time
14:00:00 - 15:00:00
Location
W4-2F-201, HKUST(GZ) & Online
Join Link
Zoom Meeting ID: 976 4751 5944
Passcode: dsat
Event Organizer
Data Science and Analytics Thrust
dsat@hkust-gz.edu.cn