GOFA: A Generative One-For-All Model for Joint Graph Language Modeling
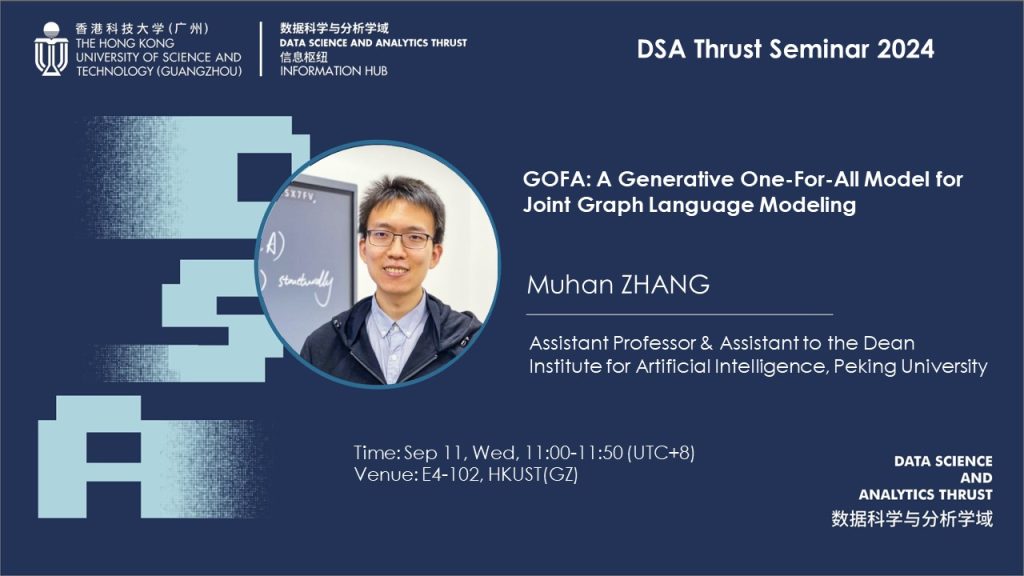
ABSTRACT
Foundation models, such as Large Language Models (LLMs) or Large Vision Models (LVMs), have emerged as one of the most powerful tools in the respective fields. However, unlike text and image data, graph data do not have a definitive structure, posing great challenges to developing a Graph Foundation Model (GFM). For example, current attempts at designing general graph models either transform graph data into a language format for LLM-based prediction or still train a GNN model with LLM as an assistant. The former can handle unlimited tasks, while the latter captures graph structure much better -- yet, no existing work can achieve both simultaneously. In this paper, we identify three key desirable properties of a GFM: self-supervised pretraining, fluidity in tasks, and graph awareness. To account for these properties, we extend the conventional language modeling to the graph domain and propose a novel generative graph language model GOFA to solve the problem. The model interleaves randomly initialized GNN layers into a frozen pre-trained LLM so that the semantic and structural modeling abilities are organically combined. GOFA is pre-trained on newly proposed graph-level next-word prediction, question-answering, and structural tasks to obtain the above GFM properties. The pre-trained model is further fine-tuned on downstream tasks to obtain task-solving ability. The fine-tuned model is evaluated on various downstream tasks, demonstrating a strong ability to solve structural and contextual problems in zero-shot scenarios.
SPEAKER BIO
Dr. Muhan Zhang is an assistant professor and assistant to the dean at Institute for Artificial Intelligence, Peking University. He is recipient of the National Excellent Youth (Overseas) Project, and Boya Young Scholar of Peking University. He graduated from the IEEE pilot class of Shanghai Jiao Tong University in 2015 and obtained his Ph.D. in Computer Science from Washington University in St. Louis in 2019. From 2019 to 2021, he was a research scientist at Facebook AI (now Meta AI). He was awarded the AI 2000 most influential scholar honorable mentions in 2022 and 2023 by Aminer. As a pioneer researcher of Graph Neural Networks, his DGCNN algorithm for graph classification was selected as one of the top ten most influential papers at AAAI-2018 and has been cited over 1500 times. His SEAL algorithm for link prediction significantly broadened the applicability of GNNs on multi-node tasks and has been cited over 2000 times. His algorithms have been written into standard libraries for graph deep learning multiple times. He regularly serves as an area chair for NeurIPS, ICML, ICLR and other top conferences, and he is a reviewer for top journals such as JMLR, TPAMI, TNNLS, TKDE, TSP, AOAS, and JAIR. He teaches Introduction to Artificial Intelligence and Machine Learning at Peking University.
Date
11 September 2024
Time
11:00:00 - 12:00:00
Location
E4-1F-102, HKUST(GZ)
Event Organizer
Data Science and Analytics Thrust
dsarpg@hkust-gz.edu.cn