Efficient Deep Neural Architecture Design and Training
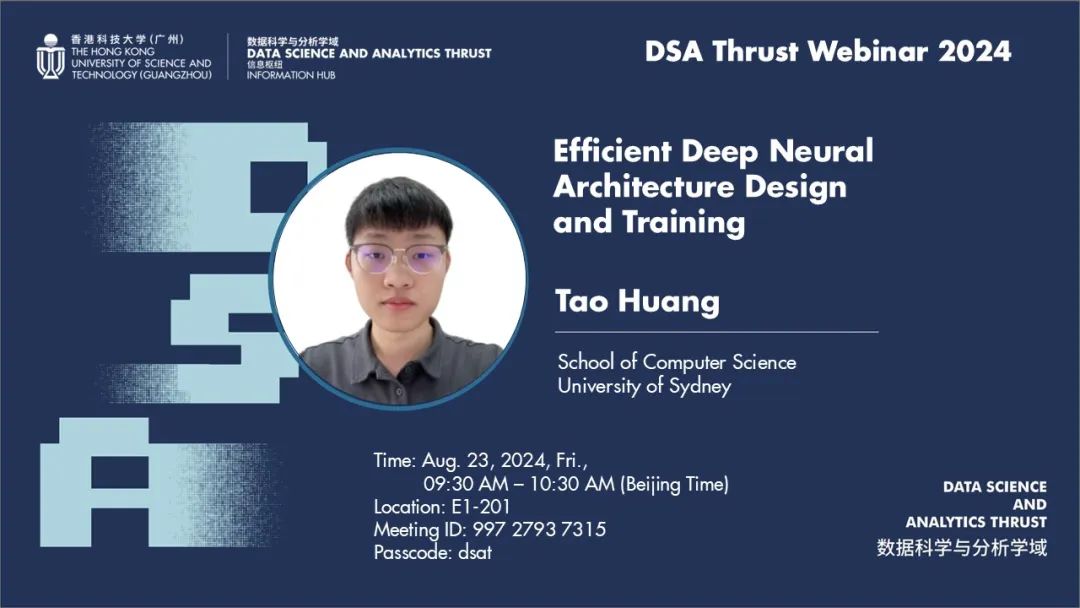
ABSTRACT
The growing complexity of machine learning models has introduced significant challenges in artificial intelligence, necessitating substantial computational resources, memory, and energy. Model compression algorithms have emerged as a critical solution in both academia and industry, forming a common pipeline for developing more efficient models. In this talk, I will present my research in two key areas of model compression: (1) Neural architecture search (NAS): I will introduce my pioneering work in enhancing the search precision and efficiency of NAS by refining the sampling strategy of architectures, leading to more optimal model designs with reduced computational overhead. (2) Knowledge distillation (KD): I will discuss my research on exploring and improving KD in the context of modern models and training strategies, addressing the unique challenges posed by the substantial capacity gap between teacher and student models. Additionally, I will briefly touch upon my investigations into the efficiency of large foundation models, highlighting emerging trends that are driving the future of efficient AI.
SPEAKER BIO
Tao HUANG
University of Sydney
Tao Huang is a final-year PhD candidate in the School of Computer Science at the University of Sydney. Prior to this, he obtained his Bachelor’s degree in Computer Science from Huazhong University of Science and Technology and worked as a Researcher at SenseTime Research. His primary research interests lie in efficient machine learning, particularly in knowledge distillation, neural architecture design, and efficient training algorithms. Tao has published over 15 papers in top-tier conferences such as CVPR, NeurIPS, ICLR, and ECCV, including 9 as the first author. He was the developer of the OpenMMLab Model Compression Toolbox - MMRazor, which integrates multiple model compression algorithms from his research and has garnered over 1,400 stars on GitHub. In industry, Tao's model compression algorithms have been integrated into SenseTime's Intelligent Cabin products. These products have established partnerships with more than 30 leading domestic and international companies, with over 13 million vehicles covered by designated mass production projects.
Date
23 August 2024
Time
09:30:00 - 10:30:00
Location
E1-2F-201, HKUST(GZ) & Online
Join Link
Zoom Meeting ID: 997 2793 7315
Passcode: dsat
Event Organizer
Data Science and Analytics Thrust
dsat@hkust-gz.edu.cn