Medical Data Management
Abstract
Our research directions include:
Medical Data Assetization Framework: The development of a medical data assetization framework is a multi-level, multi-dimensional task. It includes in-depth studies on the characteristics and structure of multimodal medical data, the development of data validation methods based on noise transition matrices and confidence checks to ensure the authenticity and reliability of data. In addition, the laboratory also focuses on improving data quality, researching data quality improvement systems based on crowdsourcing strategies and weak supervision techniques to enhance the accuracy and consistency of data, laying a solid foundation for subsequent data analysis and model training. At the same time, in terms of data privacy protection, strict security measures and policies are formulated, utilizing encryption, anonymization, and authorized access technologies to ensure the security of medical data, preventing data leaks and unauthorized access.
Medical Model Explainability System: In the construction of a medical model explainability system, the laboratory emphasizes the importance of model explanation, which is a crucial link to ensure the safety and reliability of AI technology and is key to improving the accuracy and trustworthiness of models. This includes researching technologies based on feature attribution and explanation outcomes, deeply exploring the decision-making logic and basis of models, identifying models' weaknesses and potential biases, and providing precise, personalized decision explanations for each patient. Meanwhile, the results of explanations are used to detect and correct model biases, ensuring the fairness and reliability of models.
Medical Multimodal Large Model Platform: There exists a problem of imbalance and asymmetry in medical resources supply and demand in the real world. By building a multimodal medical large model platform, the laboratory aims to integrate multi-source data to advance medical research and optimize the clinical decision-making process. This not only helps to comprehensively improve the health level of the population but also provides key technological support for China's scientific and technological innovation and industrial upgrading in the medical and health field. Specifically, by building an auxiliary diagnosis and treatment platform, it achieves efficient integration and management of multi-source data, and by researching the annotation system for medical multimodal large model data, it ensures the quality and consistency of data, providing precise, reliable data support for model training and optimization, significantly enhancing the intelligence level of medical services, offering more accurate and convenient medical services to the masses.
Project members
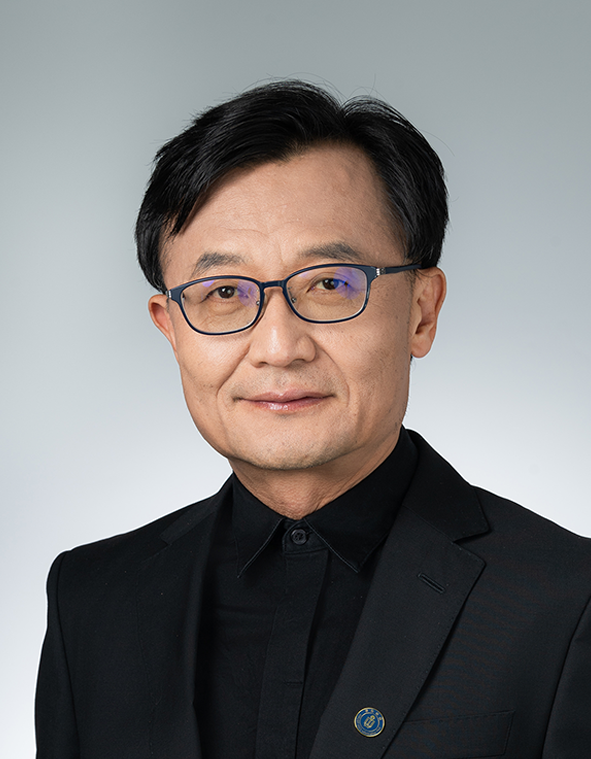
Lei CHEN
Chair Professor
Research Area
Sector-Specific Data Analytics