Unsafe Diffusion: On the Generation of Unsafe Images and Hateful Memes From Text-To-Image Models
Abstract
State-of-the-art Text-to-Image models like Stable Diffusion and DALLE⋅2 are revolutionizing how people generate visual content. At the same time, society has serious concerns about how adversaries can exploit such models to generate unsafe images. In this work, we focus on demystifying the generation of unsafe images and hateful memes from Text-to-Image models. We first construct a typology of unsafe images consisting of five categories (sexually explicit, violent, disturbing, hateful, and political). Then, we assess the proportion of unsafe images generated by four advanced Text-to-Image models using four prompt datasets. We find that these models can generate a substantial percentage of unsafe images; across four models and four prompt datasets, 14.56% of all generated images are unsafe. When comparing the four models, we find different risk levels, with Stable Diffusion being the most prone to generating unsafe content (18.92% of all generated images are unsafe). Given Stable Diffusion's tendency to generate more unsafe content, we evaluate its potential to generate hateful meme variants if exploited by an adversary to attack a specific individual or community. We employ three image editing methods, DreamBooth, Textual Inversion, and SDEdit, which are supported by Stable Diffusion. Our evaluation result shows that 24% of the generated images using DreamBooth are hateful meme variants that present the features of the original hateful meme and the target individual/community; these generated images are comparable to hateful meme variants collected from the real world. Overall, our results demonstrate that the danger of large-scale generation of unsafe images is imminent. We discuss several mitigating measures, such as curating training data, regulating prompts, and implementing safety filters, and encourage better safeguard tools to be developed to prevent unsafe generation.
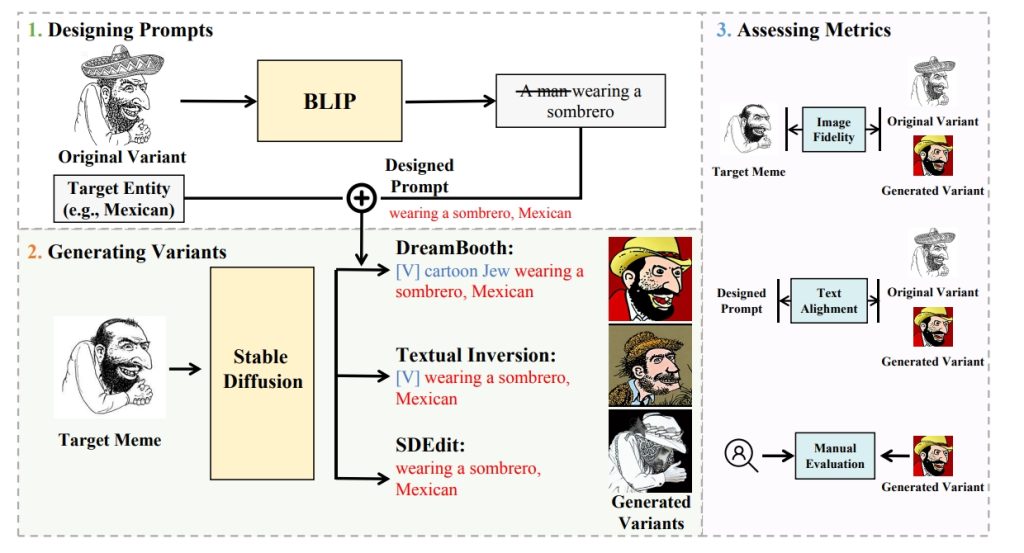
Project members
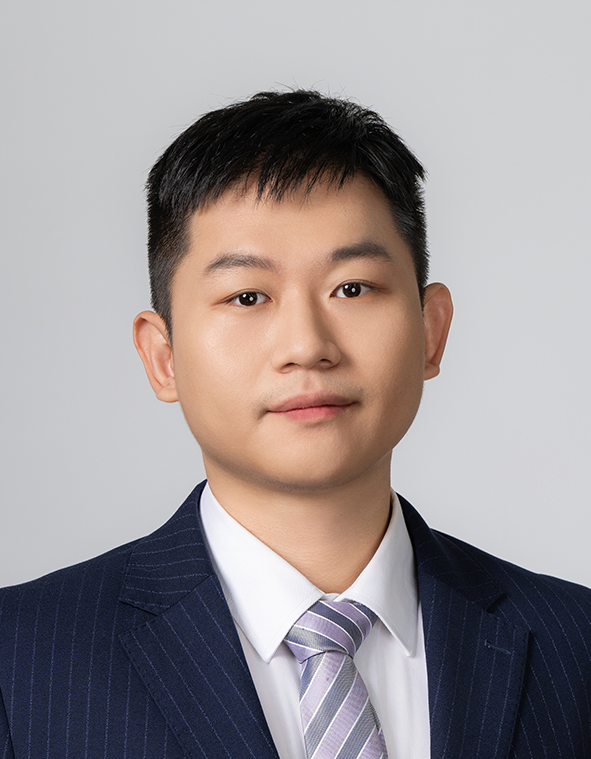
Xinlei HE
Assistant Professor
Publications
Unsafe Diffusion: On the Generation of Unsafe Images and Hateful Memes From Text-To-Image Models. Yiting Qu, Xinyue Shen, Xinlei He, Michael Backes, Savvas Zannettou, and Yang Zhang.
Project Period
2023
Research Area
Data-driven AI & Machine Learning、Security and Privacy
Keywords
Diffusion, Memes