Robust Attributed Graph Alignment via Joint Structure Learning and Optimal Transport
Abstract
Graph alignment, which aims at identifying corresponding entities across multiple networks, has been widely applied in various domains. As the graphs to be aligned are usually constructed from different sources, the inconsistency issues of structures and features between two graphs are ubiquitous in real-world applications. Most existing methods follow the "embed-then-cross-compare" paradigm which computes node embeddings in each graph and then processes node correspondences based on cross-graph embedding comparison. However, we find these methods are unstable and sub-optimal when structure or feature inconsistency appears. To this end, we propose SLOTAlign, an unsupervised graph alignment framework that jointly performs Structure Learning and Optimal Transport Alignment. We convert graph alignment to an optimal transport problem between two intra-graph matrices without the requirement of cross-graph comparison. We further incorporate multi-view structure learning to enhance graph representation power and reduce the effect of structure and feature inconsistency inherited across graphs. Moreover, an alternating scheme based algorithm has been developed to address the joint optimization problem in SLOTAlign and the provable convergence result are also established. Finally, we conduct extensive experiments on six unsupervised graph alignment datasets and the DBP15K knowledge graph (KG) alignment benchmark dataset. The proposed SLOTAlign shows superior performance and strongest robustness over seven unsupervised graph alignment methods and five specialized KG alignment methods.
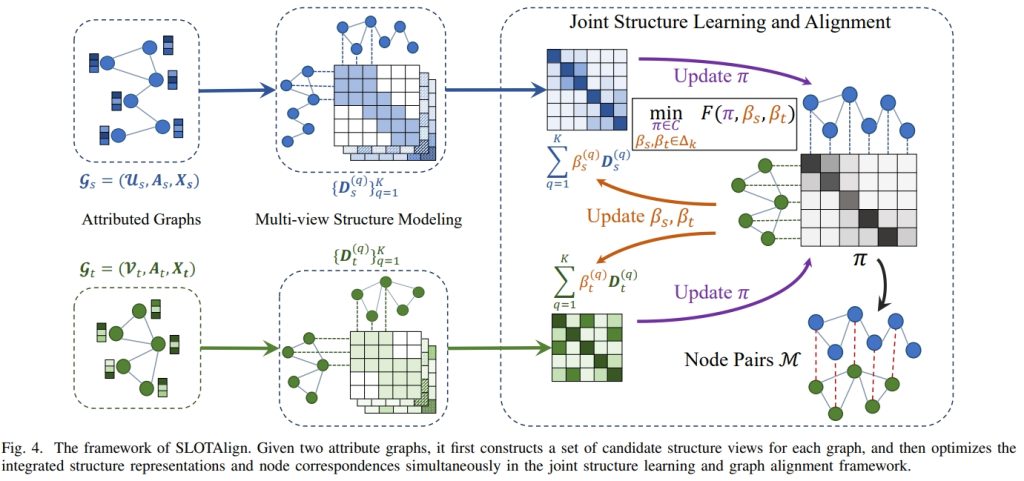
Project members
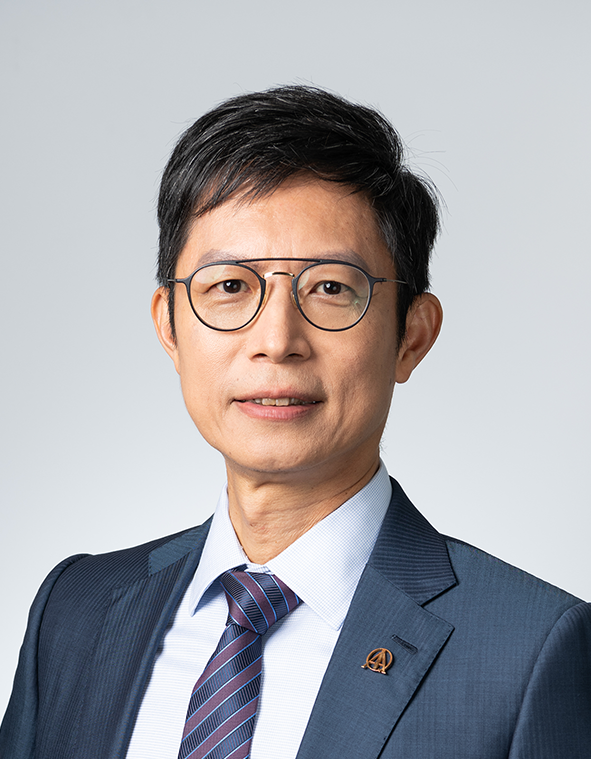
Fugee TSUNG
Chair Professor
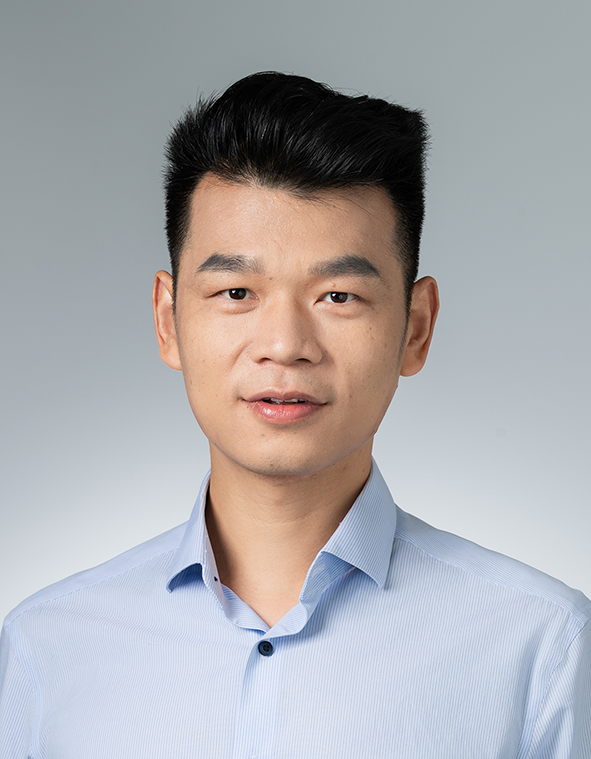
Jia LI
Assistant Professor
Publications
Robust Attributed Graph Alignment via Joint Structure Learning and Optimal Transport. Jianheng Tang, Weiqi Zhang, Jiajin Li, Kangfei Zhao, Fugee Tsung, and Jia Li.
Project Period
2023
Research Area
Data-driven AI
Keywords
Graph alignment, Optimal transport, Structure learning, Unsupervised Learning