Rethinking Graph Neural Networks for Anomaly Detection
Abstract
components for GNN design is to select a tailored spectral filter, we take the first step towards analyzing anomalies via the lens of the graph spectrum. Our crucial observation is the existence of anomalies will lead to the ‘right-shift’ phenomenon, that is, the spectral energy distribution concentrates less on low frequencies and more on high frequencies. This fact motivates us to propose the Beta Wavelet Graph Neural Network (BWGNN). Indeed, BWGNN has spectral and spatial localized band-pass filters to better handle the ‘right-shift’ phenomenon in anomalies. We demonstrate the effectiveness of BWGNN on four large-scale anomaly detection datasets. Our code and data are released at https://github.com/squareRoot3/Rethinking-Anomaly-Detection.
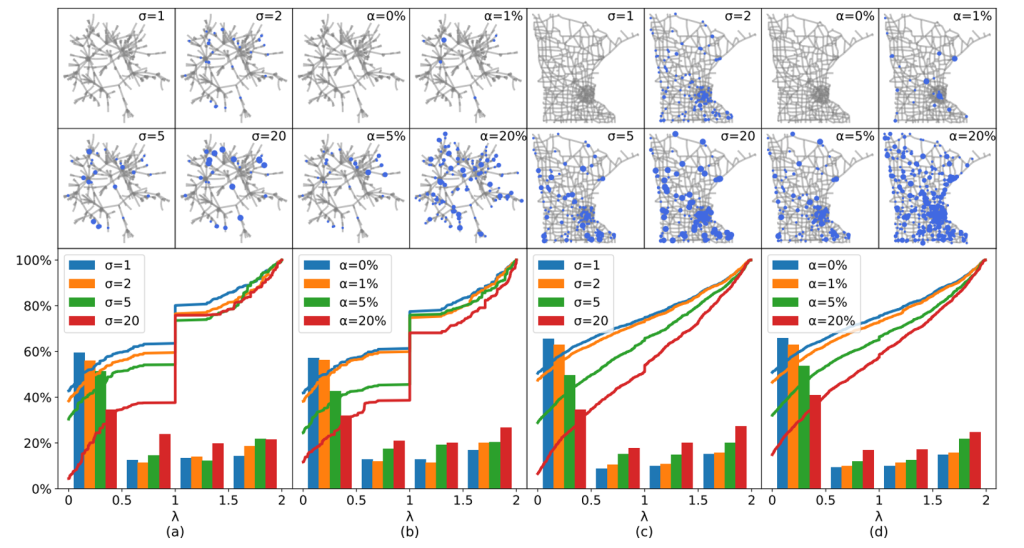
Project members
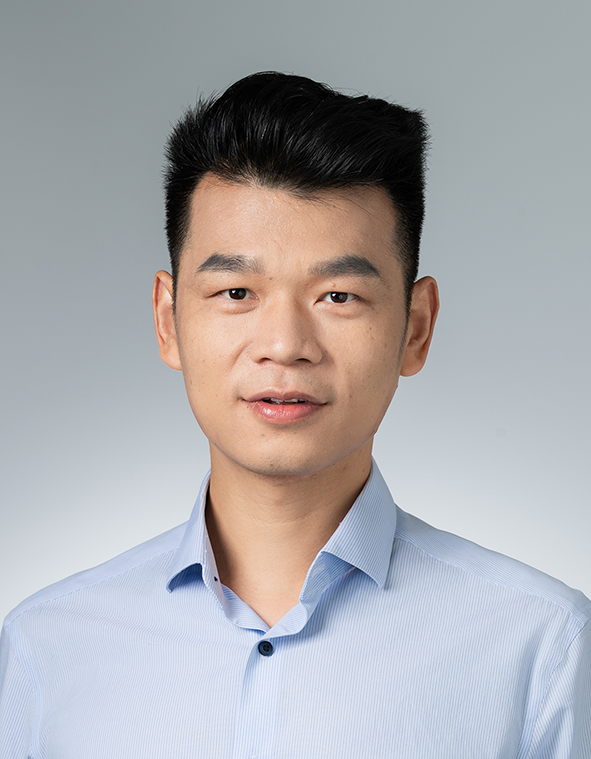
Jia LI
Assistant Professor
Publications
Rethinking Graph Neural Networks for Anomaly Detection. Jianheng Tang, Jiajin Li, Ziqi Gao, and Jia Li.
Project Period
2022
Research Area
Data-driven AI
Keywords
Anomaly Detection, graph neural networks