Reference Panel Guided 3D Genome Data Analysis
Abstract
The widespread usage of Hi-C has revealed the hierarchical structures of the genome, thereby deepening our understanding of the organization and function of 3D genomes. However, analyzing Hi-C data remains a challenging task, mainly due to the sequencing coverage of data produced in most Hi-C experiments is insufficient.
In this project, we proposed a reference panel enabled framework to tackle the data insufficiency issue in Hi-C data analysis. This pioneering approach represents the first instance of harnessing the vast amount of existing Hi-C datasets while analyzing a given study Hi-C dataset. Within this framework, we developed three applications to enhance a Hi-C contact map, annotate chromatin loops, and identify nested topologically associating domains (TADs) from insufficiently sequenced Hi-C data. Algorithms developed in this thesis leverage ideas from attention mechanisms, representation learning, dynamic programming, and non-parametric statistics. The introduction of a panel of reference Hi-C samples significantly improved prediction accuracy across three diverse Hi-C data analysis tasks under a wide spectrum of benchmarking scenarios. Applying our tools to Hi-C data from various cells deepened our understanding of the formation of TADs and chromatin loops, unraveling key insights into these essential genomic features.
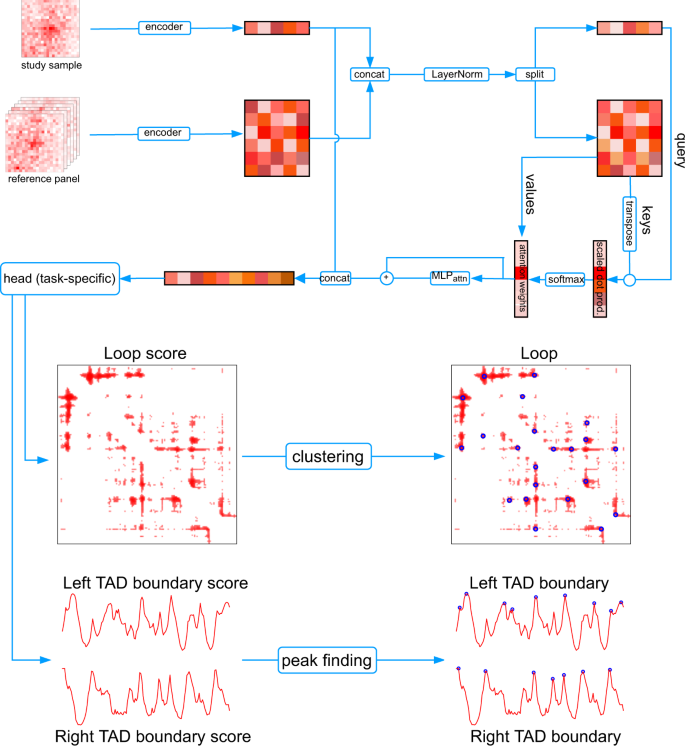
Project members
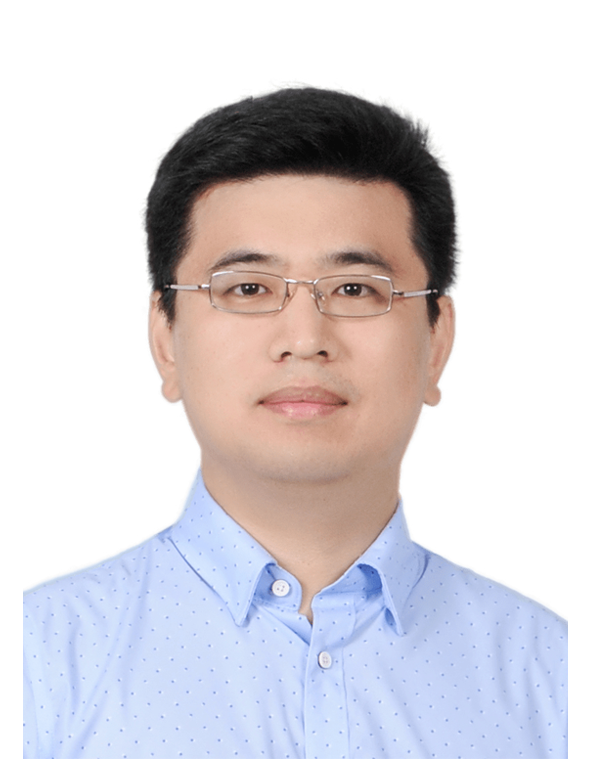
Yanlin ZHANG
Assistant Professor
Publications
1. Reference panel guided topological structure annotation of Hi-C data. Yanlin Zhang, and Mathieu Blanchette.
2. Reference panel-guided super-resolution inference of Hi-C data. Yanlin Zhang, and Mathieu Blanchette.
Project Period
2022.12.1-2023.6.30
Research Area
Computational biology
Keywords
3D genome, attention mechanism, computational biology, deep learning