A Co-training Approach for Noisy Time Series Learning
Abstract
In this work, we focus on robust time series representation learning. Our assumption is that real-world time series is noisy and complementary information from different views of the same time series plays an important role while analyzing noisy input. Based on this, we create two views for the input time series through two different encoders. We conduct co-training based contrastive learning iteratively to learn the encoders. Our experiments demonstrate that this co-training approach leads to a significant improvement in performance. Especially, by leveraging the complementary information from different views, our proposed TS-CoT method can mitigate the impact of data noise and corruption. Empirical evaluations on four time series benchmarks in unsupervised and semi-supervised settings reveal that TS-CoT outperforms existing methods. Furthermore, the representations learned by TS-CoT can transfer well to downstream tasks through fine-tuning1.
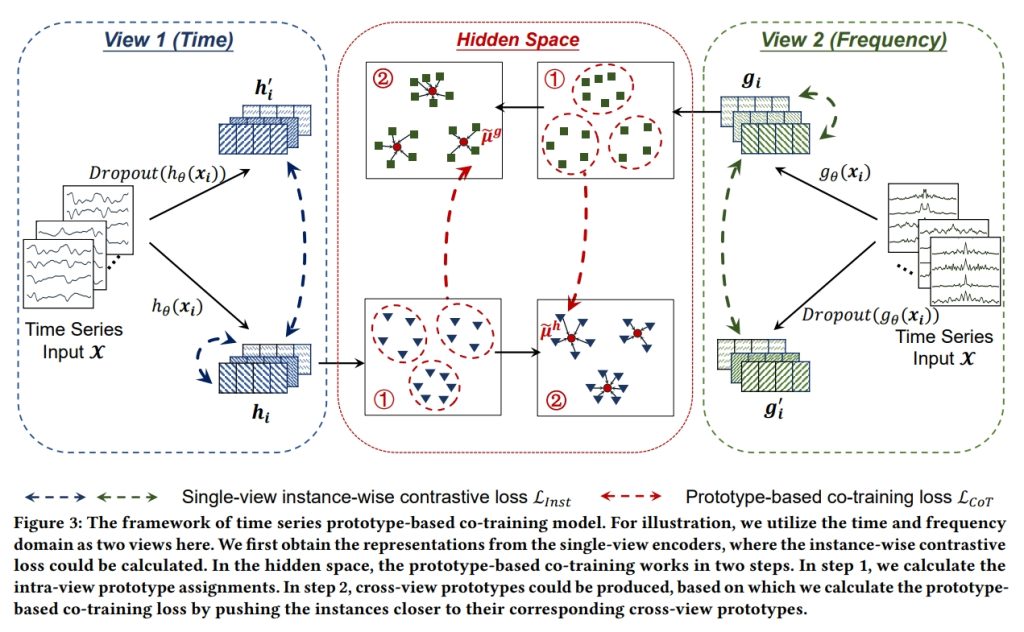
Project members
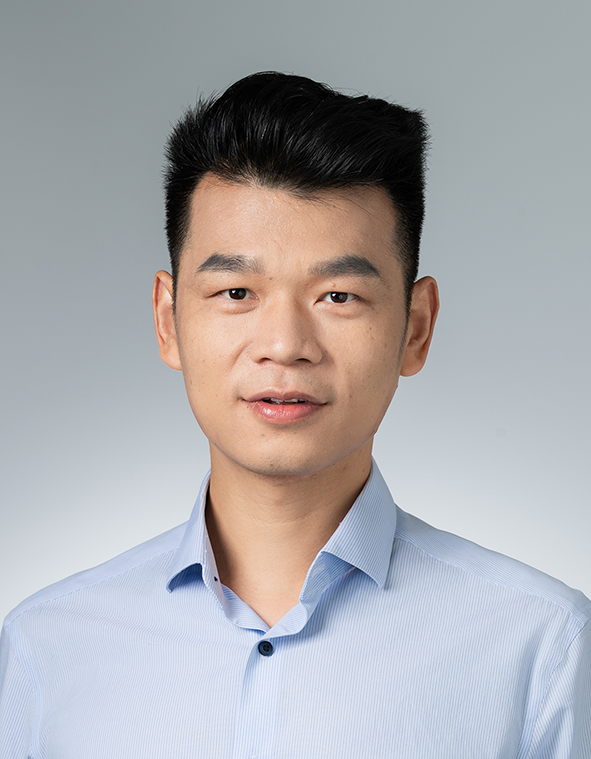
Jia LI
Assistant Professor
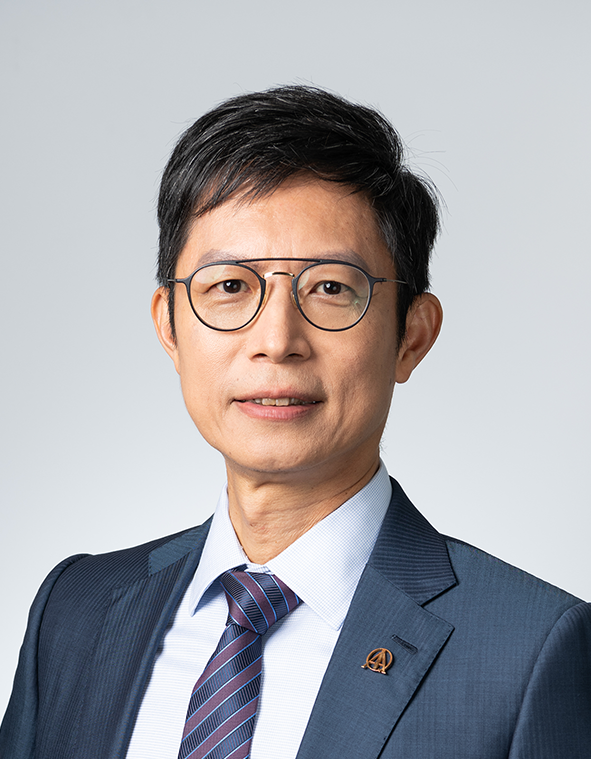
Fugee TSUNG
Chair Professor
Publications
A Co-training Approach for Noisy Time Series Learning. Weiqi Zhang, Jianfeng Zhang, Jia Li, and Fugee Tsung.
Project Period
2023
Research Area
Data-driven AI
Keywords
Co-training, Contrastive Learning, Noisy Data, Time Series