Time Series Anomaly Detection and Diagnosis Based on Deep Learning in Industrial Scenarios
Abstract
With the development of digital transformation in the industry, time series data under industrial scenarios presents characteristics of high dimensionality, large volume, and strong nonlinearity, which brings enormous challenges for industrial system anomaly monitoring, detection, and diagnosis. Deep learning-based time series anomaly detection models have been developed in recent years. However, the current research does not utilize the rich domain prior knowledge in industrial scenarios when performing anomaly monitoring and poorly models the temporal autocorrelation among time-series data. Besides, black-box models such as deep learning have low interpretability, making it challenging to accurately locate and trace anomalies in industrial deployment. This project will propose a new framework for industrial time series anomaly detection and diagnosis based on the above gaps. Specifically, the project first incorporates domain expert knowledge into anomaly measurement-oriented model design and models the temporal correlation of anomaly scores based on statistical process control to give accurate anomaly determinations. Further, the project will learn causality in deep learning models based on data augmentation strategies to achieve anomaly localization and diagnosis. The project will give a professional, efficient, stable, and interpretable solution for time series anomaly detection and diagnosis under industrial scenarios through an innovative framework design.
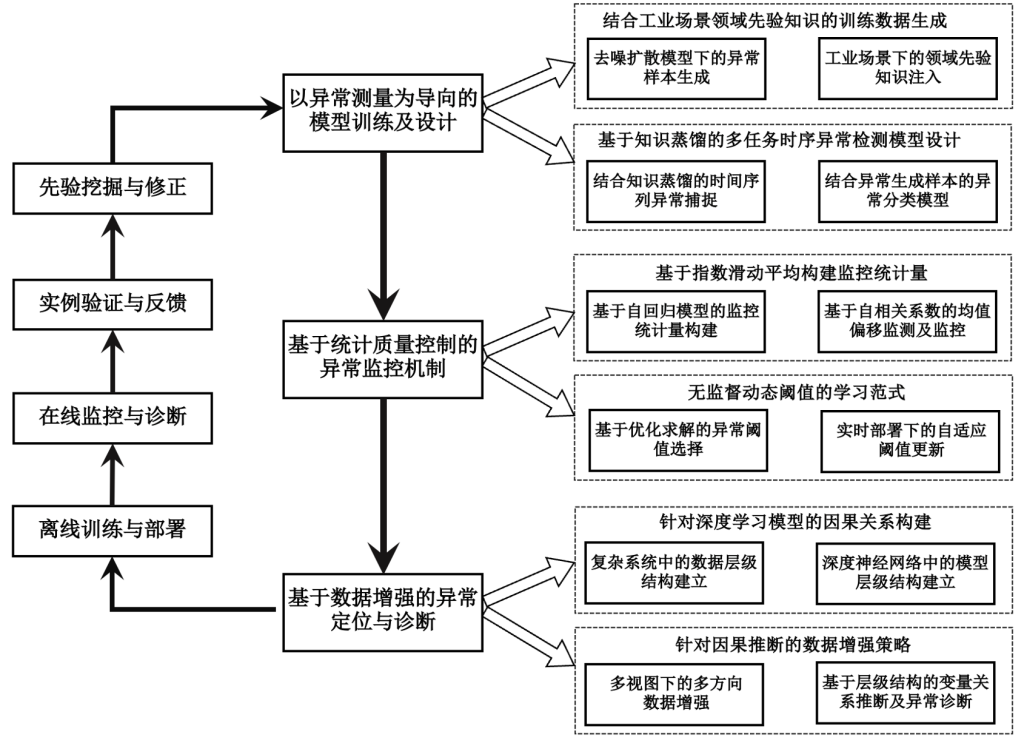
Project members
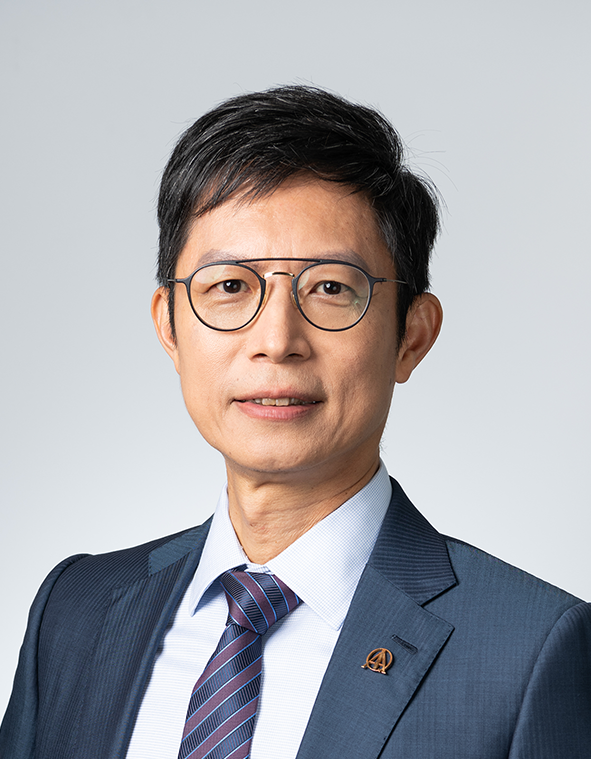
Fugee TSUNG
Chair Professor
Project Period
2024/1/1-2027/12/31
Research Area
Industrial and Business Analytics, Statistical Learning and Modeling, Data-driven AI & Machine Learning
Keywords
Data-Driven Process modeling; Industrial Data Analytics; Anomaly Detection and Diagnosis; Time Series Data Stream