Secure Self-supervised Learning
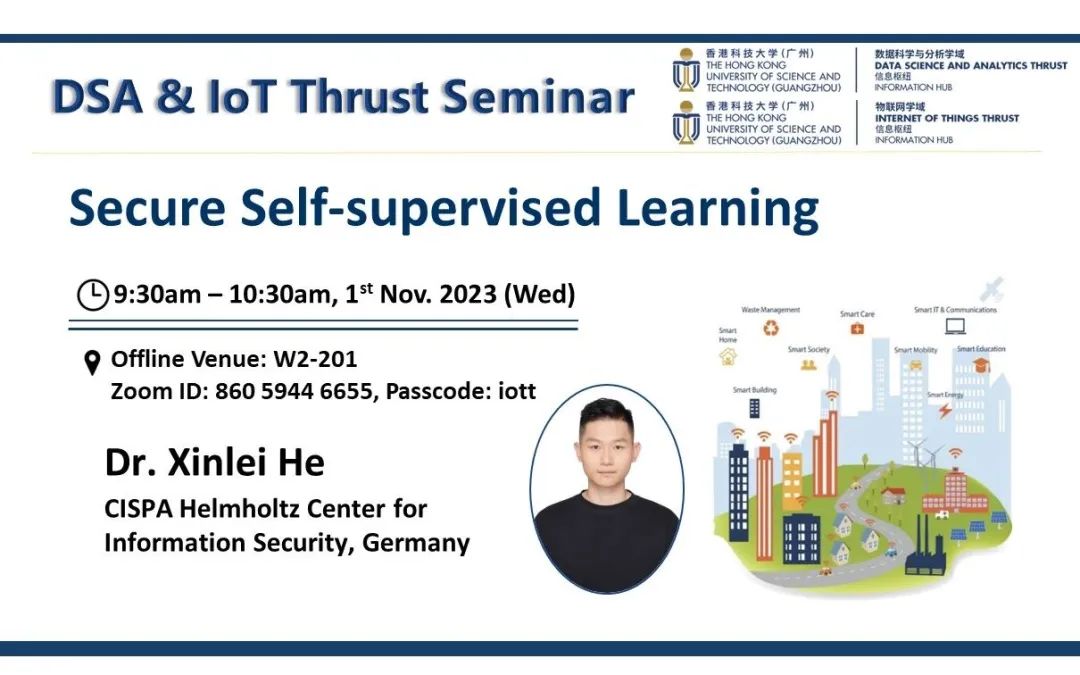
ABSTRACT
Self-supervised learning (SSL) is an emerging machine learning (ML) paradigm, which relies on unlabeled datasets to pre-train powerful encoders that can then be treated as feature extractors for various downstream tasks. Despite being powerful, SSL is also vulnerable to various security and privacy attacks. In this talk, I will summarize some of our recent works covering both attacks and defenses, with a particular focus on membership/attribute inference attacks, more effective model stealing attacks, and copyright protection. I will wrap up with a discussion of open directions on this topic.
SPEAKER BIO
Xinlei HE
CISPA Helmholtz Center for Information Security
Xinlei He obtained his Ph.D. from CISPA Helmholtz Center for Information Security. His research lies in the domain of trustworthy machine learning, with a special focus on privacy, security, and accountability issues stemming from machine learning paradigms. He has published over 20 papers in top-tier conferences/journals such as IEEE S&P, ACM CCS, and USENIX Security. He served as the TPC member of IEEE S&P 2024, ASIACCS 2024, and ESORICS 2022. He was the recipient of The Norton Labs Graduate Fellowship 2022.
More details are at https://xinleihe.github.io/.
Date
01 November 2023
Time
09:30:00 - 10:30:00
Location
W2-2F-201, HKUST(GZ)
Join Link
Zoom Meeting ID: 860 5944 6655
Passcode: iott
Event Organizer
Data Science and Analytics Thrust Internet of Things Thrust
dsat@hkust-gz.edu.cn iott@hkust-gz.edu.cn