Efficient Learning via Model Compression and Effective Learning via Dynamics-Aware Learning Strategies
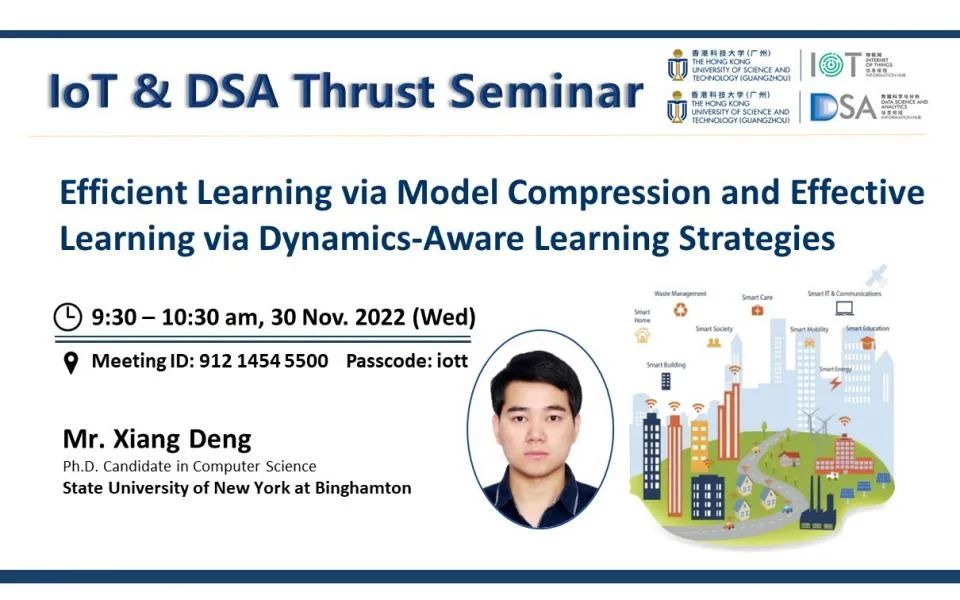
ABSTRACT
Deep neural networks (DNNs) have been successfully applied to a wide range of applications in different areas. However, the superior performances of DNNs are accompanied with large amounts of memory and computation requirements, which seriously restricts their deployment on resource-limited devices and latency-sensitive applications. To improve the efficiency, we study model compression from different perspectives. In addition to efficiency, it is always appealing to further improve the effectiveness of DNNs, thus benefiting the existing applications and extending the application horizon to more accuracy-critical or safety-critical domains. Further improving the effectiveness of a DNN usually needs to substantially, even exponentially, increase the number of its parameters. To avoid the extra cost, we propose dynamics-aware learning strategies to enhance the performance of a DNN by exploring the learning dynamics of DNNs.
SPEAKER BIO
Xiang DENG
Binghamton University
Xiang Deng is a PhD candidate in State University of New York at Binghamton. He aims to design simple yet effective deep learning approaches to address the problems in different areas. His research interests are mainly on machine learning, deep learning, and the related applications, such as model compression, model generalization, and robustness. His work has been published in international machine learning and artificial intelligence conferences and journals such as ICML, NeurIPS, ECCV, AAAI, IJCAI, and Neural Networks. He also served as PC members or reviewers of these conferences or journals.
Date
30 November 2022
Time
09:30:00 - 10:30:00
Location
Online
Join Link
Zoom Meeting ID: 912 1454 5500
Passcode: iott
Event Organizer
Data Science and Analytics Thrust Internet of Things Thrust
dsat@hkust-gz.edu.cn iott@hkust-gz.edu.cn