An overview of Graph Generative Models and Their Applications on Molecular Generation
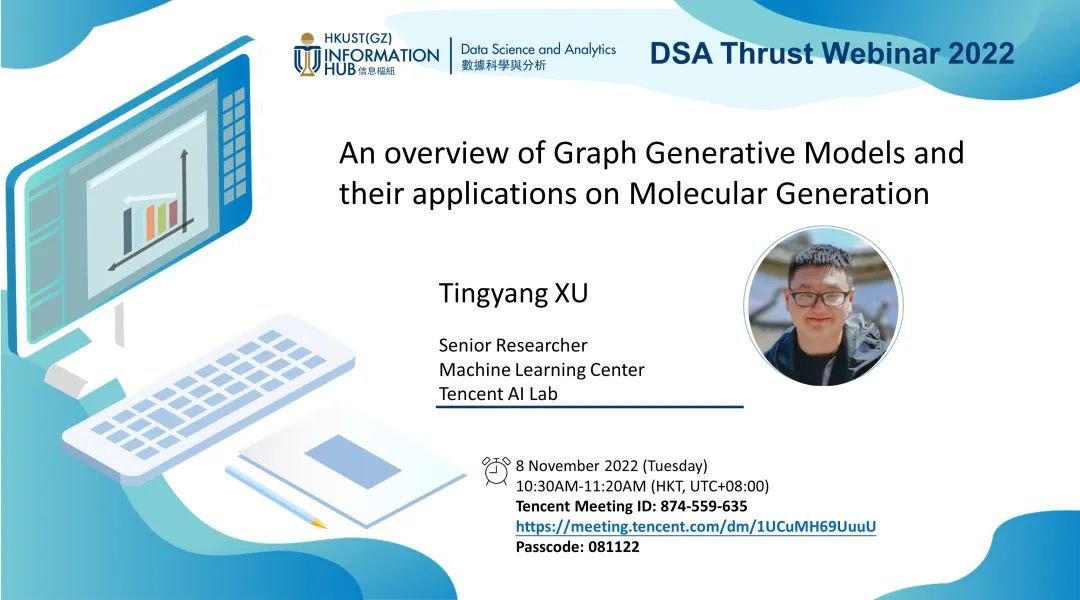
ABSTRACT
Generating novel graph structures is a fundamental problem for studies in drug discovery, engineering, and social networks. Although a set of different generative models, such as VAE, GAN, Normalizing Flow, and diffusion models, have achieved great successes in image processing, it is still hard to naively apply such generative models directly to graph structures due to the complex distribution, non-unique nature of graph and non-local dependencies between nodes and edges in a graph.
In this report, we will review how above generative models are employed into graph generations and their proper applications on the task of molecular generation. We will introduce VGAE, JTVAE, and GraphGMVAE as VAE-based methods; MolGAN and MolAICal as GAN-based methods; MoFlow and GraphDF as Flow-based methods. We will also discuss the current challenges for diffusion models in graph generation.
SPEAKER BIO
Tingyang XU
Senior Researcher
Tencent AI Lab
Tingyang Xu is a Senior researcher of Machine Learning Center in Tencent AI Lab. He obtained the Ph.D. degree from The University of Connecticut in 2017 and the bachelor’s degree from Shanghai Jiaotong University. In Tencent AI Lab, he is working on deep graph learning, graph generations and applying the deep graph learning model to various applications, such as molecular generation and rumor detection. His main research interests include social network analysis, graph neural networks, and graph generations, with particular focus on designing deep and complex graph learning models for molecular generations. He has published several papers on data mining, machine learning top conferences KDD, WWW, NeurIPS, ICLR, CVPR, ICML, etc.
Date
08 November 2022
Time
10:30:00 - 11:20:00
Location
Online
Join Link
Tencent Meeting ID:
874-559-635
Passcode: 081122
Event Organizer
Data Science and Analytics Thrust
dsat@hkust-gz.edu.cn