AI Research in Industry—Some Topics that are Deprecated, Being Depreciated, and To be Depreciated
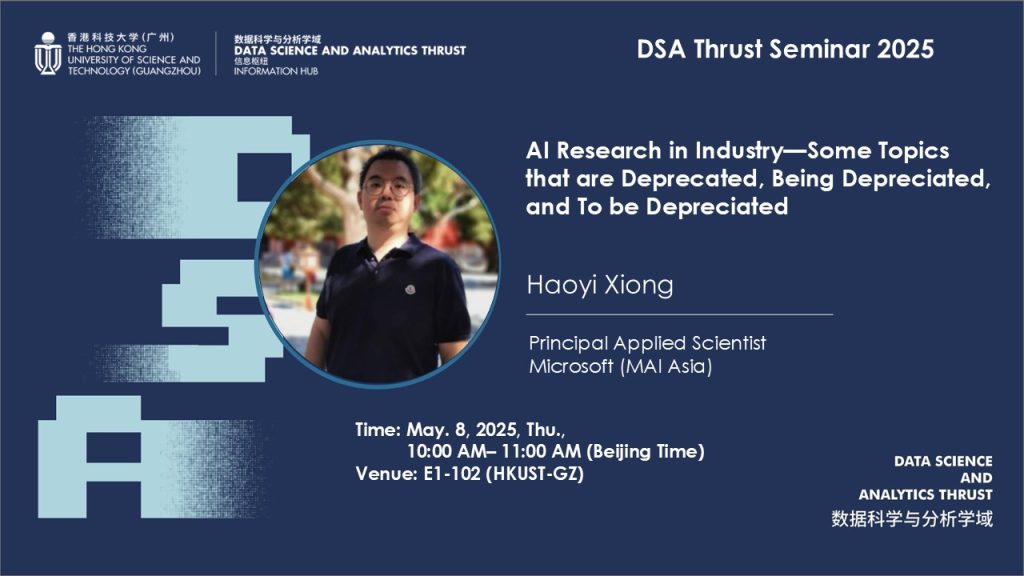
ABSTRACT
This talk provides a reflective journey through my AI research from 2019 to 2025, organized into three focal areas and examined through the lens of three essential scaling laws: Moore’s law, model scaling law, and a new AI infrastructure refresh law. First, we revisit data-driven automated deep learning—including neural architecture search, transfer learning, and domain adaptation—which formed the bedrock of early industrial AI innovations but have since become deprecated. Their limitations in scalability and adaptability, highlighted by the diminishing returns of simply increasing model capacity or algorithmic complexity, serve as a case study in the boundaries of traditional scaling laws. Next, we explore foundation model-based web search and recommendation systems that build on pre-trained models. These methods initially yielded rapid improvements in handling web-scale data and personalization tasks, yet they are now being depreciated. While traditional model scaling laws suggest performance gains with increased parameters, the interplay with hardware constraints has exposed practical cost and efficiency bottlenecks. Finally, we turn our attention to the emerging frontier of pre-training and post-training of foundation models with applications to sciences and interdisciplinary exploration. These methods, designated as to be depreciated, are assessed using a new scaling perspective—the AI Infrastructure Refresh Law. This law emphasizes that each new release of advanced AI chips forces AI companies into a strategic gamble: they must invest aggressively enough in the latest hardware (higher AI capabilities per unit cost) to dilute expensive legacy chips but must avoid overinvestment that could jeopardize future upgrades. However, the resulting weak profit margins render this research type economically unsustainable and fail to justify continuous investment in rapidly advancing hardware cycles. The material of this talk is derived from my research outcome and publications in Baidu. Opinions are my own.
SPEAKER BIO
Dr. Haoyi Xiong is now a principal applied scientist at Microsoft (MAI Asia). Previously, he was the principal architect and research manager at the Big Data Laboratory, Baidu Research. From 2016 to 2018, he served as a tenure-track assistant professor in the Computer Science Department at Missouri S&T and was a research associate at the University of Virginia in 2015-2016. By courtesy, Dr. Xiong has also served the University of Central Florida as a graduate faculty scholar in the ECE PhD program since 2019. He earned his Ph.D. in electrical and computer engineering in 2015 from Télécom SudParis and Université Pierre-et-Marie-Curie, under the supervision of Prof. Daqing Zhang. His research interests include ubiquitous computing, machine learning, and data sciences. He has published intensively in the flagship venues, including Nature Machine Intelligence, UbiComp, KDD, ICML, NeurIPS, ICLR, JMLR/TMLR, Machine Learning, AAAI/IJCAI, ICDM, and various IEEE/ACM Transactions. He is the co-recipient of several awards, including ICDM’23 Best Student Paper Award, DSAA’23 Best Paper Awards Journal Track, IEEE TCSC Awards for Early Career Researchers (2020), and the First Prize of Science and Technology Advancements Award, Chinese Institute of Electronics (2019). Dr. Xiong is a Senior Member of IEEE and a Fellow of IET.
Date
08 May 2025
Time
10:00:00 - 11:00:00
Location
E1-102 (HKUST-GZ)
Event Organizer
Data Science and Analytics Thrust
dsat@hkust-gz.edu.cn