When Graph meets Large Models
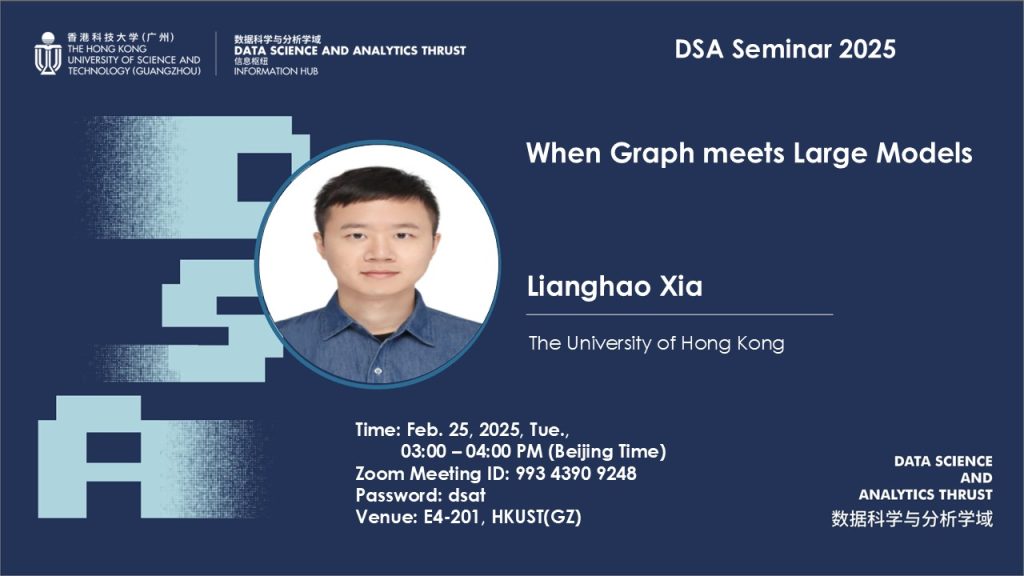
ABSTRACT
How can we empower AI systems to truly understand and reason about complex relational data in the era of Large Language Models? While LLMs have revolutionized AI, they face fundamental limitations in processing structured information and understanding intricate knowledge dependencies. My research addresses this critical challenge through Graph Foundation Models (GFMs). In this talk, I will first present our pioneering work on GFMs that effectively capture and reason about complex graph-structured information. Through novel large-scale model building techniques, we achieve robust and generalizable graph learning capabilities previously unattainable. I will demonstrate how our framework overcomes traditional limitations in processing massive-scale graph structures while preserving crucial topological information. Then, I will showcase how our graph learning frameworks significantly enhance LLMs' reasoning capabilities by integrating graph-structured knowledge through Retrieval-Augmented Generation (RAG) and Autonomous Agents. This powerful synergy between GFMs and LLMs enables sophisticated reasoning over structured data, with applications spanning recommendations, biology, urban computing, and AI agents. Our work opens new horizons for foundation models, marking a significant step toward more capable and generalizable AI systems.
SPEAKER BIO
Dr. Lianghao Xia is currently a Research Assistant Professor in the School of Computing and Data Science at the University of Hong Kong. His research primarily focuses on foundation models, large language models, and graph learning, exploring their applications in recommender systems, and spatio-temporal modeling. Since 2020, Dr. Xia has published 15 first-authored papers in leading conferences and journals including KDD, SIGIR, WWW, AAAI, ICDE, TKDE, etc. His work has been recognized with 11 Most Influential Papers, 3 Best Paper Nominations, and a number of Top 10% Most-Cited Papers, accumulating over 4,500 citations, including over 2,500 citations in 2024 alone. As a key contributor, his open-sourced project has garnered over 15k stars on GitHub. He is honored to be listed among Stanford University’s World’s Top 2% Scientists in 2024, and in Aminer’s AI 2000 Most Influential Scholars (2022-2023) in Data Mining and Information Retrieval.
Date
25 February 2025
Time
15:00:00 - 16:00:00
Location
E4-201,HKUST(GZ)
Join Link
Zoom Meeting ID: 993 4390 9248
Passcode: dsat
Event Organizer
Data Science and Analytics Thrust
dsat@hkust-gz.edu.cn