Advancing Small Language Models as Efficient Reasoners
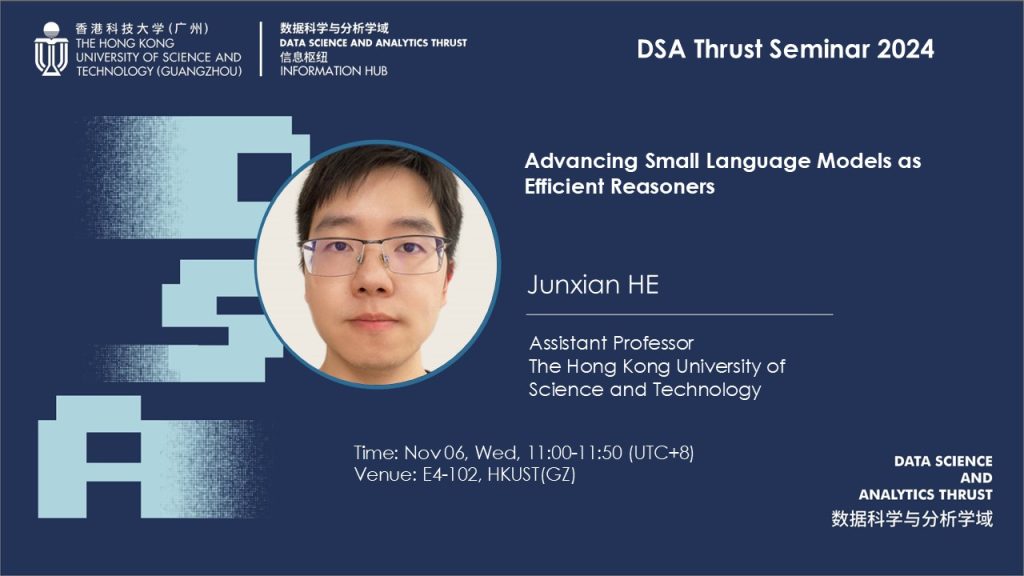
ABSTRACT
Complex reasoning tasks, such as mathematical reasoning, coding, and planning, present significant challenges for language models, particularly small, open models. In this talk, I will cover our recent research on advancing open, small language models as efficient reasoners for complex tasks. First, I will introduce DART-Math, a new data synthesis method and accompanied datasets for mathematical reasoning, achieving state-of-the-art COT reasoning performance. Then, I will present B-STaR, our innovative self-improving algorithm that monitors training dynamics and balances exploration and exploitation to achieve scalable improvements for self-taught reasoners. I will also discuss our research on non-myopic generation, which enhances the performance of language models across various complex reasoning scenarios at decoding time. Lastly, I will present our exaimination for the "math for AI" vision, studying whether learning mathematical problem-solving, a highly popular task recently, can help LLMs learn general reasoning or not.
SPEAKER BIO
Junxian He is an assistant professor in the department of computer science and engineering at the Hong Kong University of Science and Technology. He received his PhD degree from Carnegie Mellon University, Language Technologies Institute. He serves as the area chair of ICLR, ACL, and EMNLP. His recent research focuses on complex reasoning/planning, mechanistic interpretation, and multimodal understanding of large language models.
Date
06 November 2024
Time
11:00:00 - 11:50:00
Location
E4-102, HKUST(GZ)
Event Organizer
Data Science and Analytics Thrust
dsarpg@hkust-gz.edu.cn