Privacy Preserved and Efficient Bayesian Network Training and Inference
Abstract
Bayesian networks (BNs) are a widely used graphical model in machine learning for representing knowledge with uncertainty, and have good interpretability. BNs can perform casual inference based on partial evidence, and are useful for medical diagnosis. The learning and inference of BNs is expensive, especially for large networks, and meanwhile, the privacy of training data needs to be preserved for critical applications in domains like medical and finance. This project will research on designing efficient and privacy preserved algorithms for BNs by exploiting GPUs and CPUs.
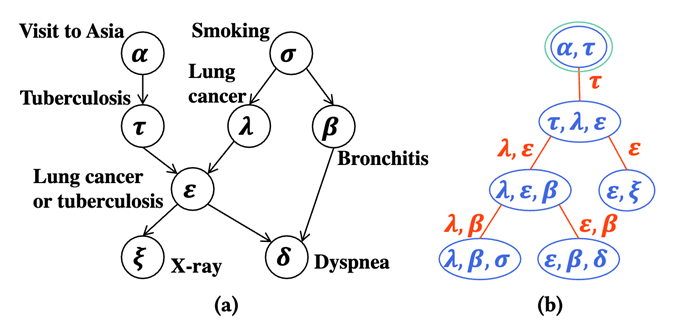
Project members
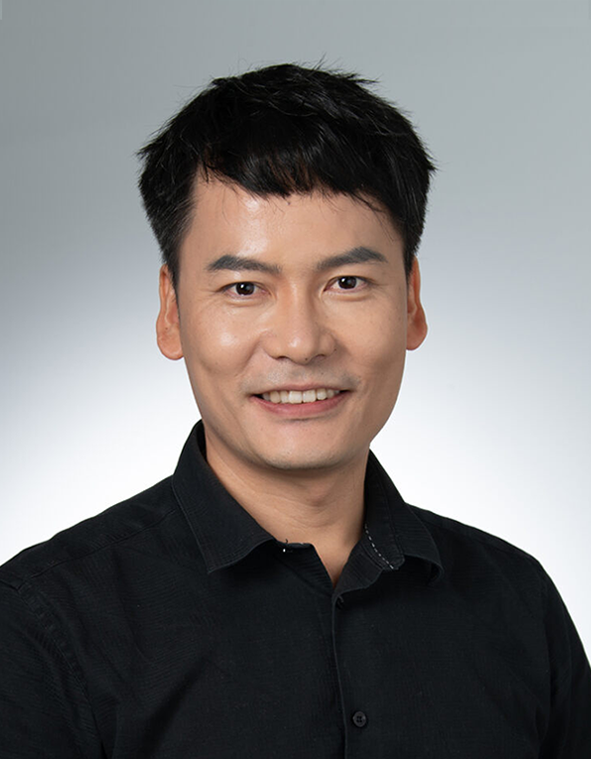
Zeyi WEN
Assistant Professor
Project Period
2023-Present
Research Area
Data-driven AI & Machine Learning、High-Performance Systems for Data Analytics