Online Learning and Optimization in Communication Networks
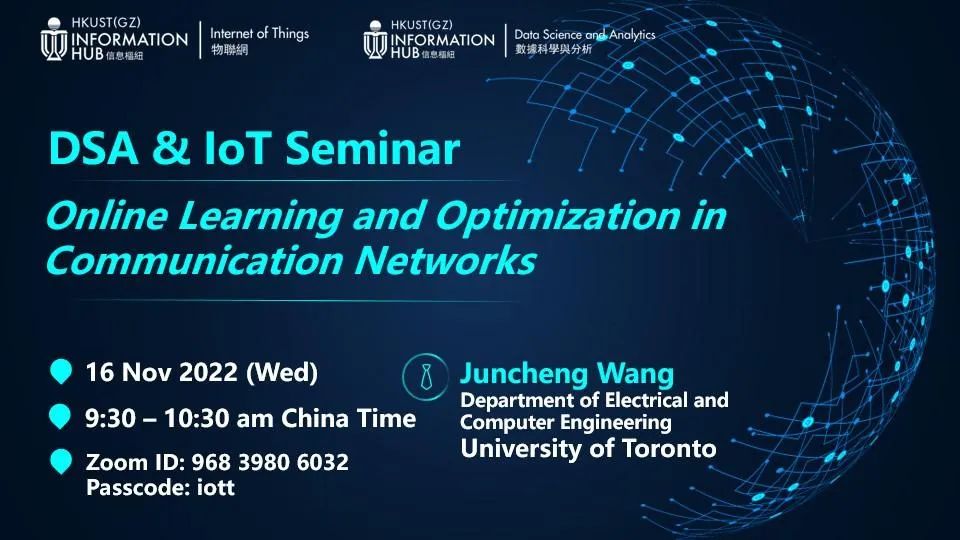
ABSTRACT
In modern wireless networks, e.g., Internet-of-Things and Vehicle-to-Vehicle systems, a large number of diverse devices communicate over radio channels. Furthermore, modern wireless networks support a wide range of computation and communication applications, e.g., augmented reality and automatic drive. Their large size and complex patterns render it difficult to employ conventional optimization techniques, while the recent great interest in machine learning proves to be opportune. Machine learning in these applications will involve large-scale computation with big data that can easily overburden conventional central servers. Distributed optimization alleviates the heavy computation loads at central servers. However, the migration of optimization from central servers to local devices will lead to a huge amount of communication overhead between them. This calls for communication-efficient distributed optimization approaches that integrate techniques from both machine learning and wireless communications.
Most prior works separately considered machine learning and wireless communications, i.e., communication designs come only after the machine learning jobs are already processed. However, since communication efficiency is strongly dependent on the computation outcomes being transmitted, the computation performance can be significantly improved by proactively optimizing communication, and vice versa. Meanwhile, online optimization is often desirable to enable adaptive learning and communication over time, due to the random nature of computation tasks and communication environment. In this talk, I will discuss how online distributed optimization can be leveraged to jointly optimize computation and communication for machine learning over wireless networks. I will also introduce some other online optimization paradigms for communication networks that accommodate feedback delay, periodic updates, and network heterogeneity in information timeliness.
SPEAKER BIO
Juncheng WANG
University of Toronto
Juncheng Wang is currently a Ph.D. candidate in the Department of Electrical and Computer Engineering at the University of Toronto, Toronto, ON, Canada. He received the B.Eng. degree in Electrical Engineering from Shanghai Jiao Tong University, Shanghai, China, in 2014, and the M.Sc. degree in Electrical and Computer Engineering from the University of Alberta, Edmonton, AB, Canada, in 2017. He worked as an Internet-of-Things engineer in Canadian industry from 2017 to 2018. His research interests include online learning, stochastic optimization, queueing theory, and their applications to computation and communication networks, such as communication-efficient distributed machine learning, network resource allocation, and wireless network virtualization.
Date
16 November 2022
Time
09:30:00 - 10:30:00
Location
Online
Join Link
Zoom Meeting ID: 968 3980 6032
Passcode: iott
Event Organizer
Data Science and Analytics Thrust Internet of Things Thrust
dsat@hkust-gz.edu.cn iott@hkust-gz.edu.cn